Download this notebook from github.
Advanced explanation for RadarSat2
Contrary to Sentinel-1, RadarSat-2 doesn’t have the notion of multi dataset
[1]:
import xsar
import geoviews as gv
import holoviews as hv
import geoviews.feature as gf
hv.extension('bokeh')
path = xsar.get_test_file('RS2_OK135107_PK1187782_DK1151894_SCWA_20220407_182127_VV_VH_SGF')
/home/datawork-cersat-public/cache/project/mpc-sentinel1/workspace/mamba/envs/testgeoviewsnumpy/lib/python3.11/site-packages/dask/dataframe/__init__.py:42: FutureWarning:
Dask dataframe query planning is disabled because dask-expr is not installed.
You can install it with `pip install dask[dataframe]` or `conda install dask`.
This will raise in a future version.
warnings.warn(msg, FutureWarning)
Access metadata from a product
Raw information is stocked in different files such as tiff ones (for digital numbers). A file named product.xml is constitued of the main information (geolocation grid, orbit attitude, noise look up tables…). Calibration look up tables are located in xml files. All the useful data is grouped in a datatree thanks to dependencie xradarsat2. This datatree is than used as an attribute of RadarSat2Meta.
[2]:
#Instanciate a RadarSat2Meta object
rs2meta = xsar.RadarSat2Meta(name=path)
/home/datawork-cersat-public/cache/project/mpc-sentinel1/workspace/mamba/envs/testgeoviewsnumpy/lib/python3.11/site-packages/xradarsat2/radarSat2_xarray_reader.py:299: UserWarning: no explicit representation of timezones available for np.datetime64
timestamp.append(np.datetime64(value["timeStamp"]).astype("datetime64[ns]"))
/home/datawork-cersat-public/cache/project/mpc-sentinel1/workspace/mamba/envs/testgeoviewsnumpy/lib/python3.11/site-packages/xradarsat2/radarSat2_xarray_reader.py:462: UserWarning: no explicit representation of timezones available for np.datetime64
timestamp.append(np.datetime64(value["timeStamp"]).astype("datetime64[ns]"))
/home/datawork-cersat-public/cache/project/mpc-sentinel1/workspace/mamba/envs/testgeoviewsnumpy/lib/python3.11/site-packages/xradarsat2/radarSat2_xarray_reader.py:570: UserWarning: no explicit representation of timezones available for np.datetime64
times.append(np.datetime64(value["timeOfDopplerCentroidEstimate"]).astype("datetime64[ns]"))
/home/datawork-cersat-public/cache/project/mpc-sentinel1/workspace/mamba/envs/testgeoviewsnumpy/lib/python3.11/site-packages/xradarsat2/radarSat2_xarray_reader.py:2056: UserWarning: no explicit representation of timezones available for np.datetime64
final_dic[key] = np.datetime64(dic[key]).astype("datetime64[ns]")
[3]:
#Access the datatree extracted from the reader
rs2meta.dt
[3]:
<xarray.DatasetView> Size: 0B Dimensions: () Data variables: *empty* Attributes: product_path: /home1/scratch/agrouaze/xsardatasync/xsardata/RS2... satellite: RADARSAT-2 inputDatasetId: /Fred/RSAT-2/610044P rawDataStartTime: 2022-04-07T18:21:27.688416000 satelliteHeight_units: m satelliteHeight: 800612.0083192665 passDirection: Descending
- timeStamp: 11
- timeStamp(timeStamp)datetime64[ns]2022-04-07T18:21:27.401672 ... 2...
array(['2022-04-07T18:21:27.401672000', '2022-04-07T18:21:35.064601000', '2022-04-07T18:21:42.727530000', '2022-04-07T18:21:50.390460000', '2022-04-07T18:21:58.053389000', '2022-04-07T18:22:05.716318000', '2022-04-07T18:22:13.379247000', '2022-04-07T18:22:21.042176000', '2022-04-07T18:22:28.705106000', '2022-04-07T18:22:36.368035000', '2022-04-07T18:22:44.030964000'], dtype='datetime64[ns]')
- yaw(timeStamp)float643.678 3.667 3.659 ... 3.581 3.562
- units :
- deg
- xpath :
- /product/sourceAttributes/orbitAndAttitude/attitudeInformation/attitudeAngles/yaw
- Description :
- Spacecraft yaw angle (units = deg). Positive for nose right rotation about the z axis (i.e. clockwise when looking along the z axis). When Right-Looking, a small positive yaw generally means that the SAR antenna is pointing slightly backwards. When Left-Looking, a small positive yaw generally means it is pointing slightly forwards.
array([3.67765042, 3.66717625, 3.65942538, 3.64531175, 3.63299634, 3.62040748, 3.61178023, 3.60112808, 3.5906719 , 3.58090494, 3.56198898])
- roll(timeStamp)float64-29.8 -29.8 -29.8 ... -29.8 -29.8
- units :
- deg
- xpath :
- /product/sourceAttributes/orbitAndAttitude/attitudeInformation/attitudeAngles/roll
- Description :
- Spacecraft roll angle (units = deg). Positive for left side up rotation about the x axis (i.e. clockwise when looking along the x axis). Roll is negative for Right-Looking and positive for Left-Looking.
array([-29.8026275 , -29.8008087 , -29.80446062, -29.8055 , -29.8016028 , -29.79519935, -29.79506787, -29.7979482 , -29.80118764, -29.80025088, -29.79991269])
- pitch(timeStamp)float64-0.002003 -0.0003909 ... -0.001624
- units :
- deg
- xpath :
- /product/sourceAttributes/orbitAndAttitude/attitudeInformation/attitudeAngles/pitch
- Description :
- Spacecraft pitch angle (units = deg). Positive for nose up rotation about the y axis (i.e. clockwise when looking along the y axis).
array([-0.00200347, -0.00039085, -0.00467112, -0.0033236 , -0.00128973, 0.00014758, -0.00418872, -0.00425894, -0.00493375, -0.00499123, -0.00162387])
- xPosition(timeStamp)float64-6.653e+06 -6.632e+06 ... -6.42e+06
- units :
- m
- xpath :
- /product/imageAttributes/geographicInformation/geolocationGrid/imageTiePoint/stateVector/xPosition
- Description :
- (units = m)
array([-6653406.85785607, -6632011.72209174, -6610187.41538696, -6587935.6440859 , -6565258.00912605, -6542155.57507657, -6518630.37436722, -6494683.60325393, -6470317.51295421, -6445533.39145136, -6420333.0962503 ])
- yPosition(timeStamp)float641.034e+06 1.043e+06 ... 1.126e+06
- units :
- m
- xpath :
- /product/imageAttributes/geographicInformation/geolocationGrid/imageTiePoint/stateVector/yPosition
- Description :
- (units = m)
array([1033831.83554106, 1043489.20598069, 1053056.57779533, 1062532.90469045, 1071917.12401067, 1081208.16934229, 1090405.02030899, 1099506.52926137, 1108511.59959665, 1117419.48739412, 1126228.81609326])
- zPosition(timeStamp)float64-2.479e+06 -2.532e+06 ... -3e+06
- units :
- m
- xpath :
- /product/imageAttributes/geographicInformation/geolocationGrid/imageTiePoint/stateVector/zPosition
- Description :
- (units = m)
array([-2479415.41166767, -2532262.13585845, -2584948.0986012 , -2637469.66542779, -2689823.69039908, -2742006.69696019, -2794015.57314536, -2845846.87916092, -2897497.26875316, -2948963.70920172, -3000242.74537346])
- xVelocity(timeStamp)float642.764e+03 2.82e+03 ... 3.316e+03
- units :
- m/s
- xpath :
- /product/imageAttributes/geographicInformation/geolocationGrid/imageTiePoint/stateVector/xVelocity
- Description :
- (units = m/s)
array([2763.95730707, 2820.0484796 , 2875.94545209, 2931.64502427, 2987.14307326, 3042.4364157 , 3097.52119183, 3152.39354088, 3207.05004241, 3261.48723633, 3315.70149995])
- yVelocity(timeStamp)float641.266e+03 1.254e+03 ... 1.143e+03
- units :
- m/s
- xpath :
- /product/imageAttributes/geographicInformation/geolocationGrid/imageTiePoint/stateVector/yVelocity
- Description :
- (units = m/s)
array([1266.09239776, 1254.42208752, 1242.61059529, 1230.65867315, 1218.56751031, 1206.3380901 , 1193.97141416, 1181.46846028, 1168.83016818, 1156.05786562, 1143.15242659])
- zVelocity(timeStamp)float64-6.907e+03 ... -6.679e+03
- units :
- m/s
- xpath :
- /product/imageAttributes/geographicInformation/geolocationGrid/imageTiePoint/stateVector/zVelocity
- Description :
- (units = m/s)
array([-6906.77410476, -6885.99045794, -6864.77024034, -6843.1146525 , -6821.02546033, -6798.50404576, -6775.55188668, -6752.17053985, -6728.36180567, -6704.12638433, -6679.46673972])
- attitudeDataSource :
- Downlink
- attitudeOffsetsApplied :
- true
- Description :
- Attitude Information Data Store. Orbit Information Data Store. State Vector Data Store. Earth Centered Rotating (ECR) coordinates.
<xarray.DatasetView> Size: 880B Dimensions: (timeStamp: 11) Coordinates: * timeStamp (timeStamp) datetime64[ns] 88B 2022-04-07T18:21:27.401672 ... ... Data variables: yaw (timeStamp) float64 88B 3.678 3.667 3.659 ... 3.591 3.581 3.562 roll (timeStamp) float64 88B -29.8 -29.8 -29.8 ... -29.8 -29.8 -29.8 pitch (timeStamp) float64 88B -0.002003 -0.0003909 ... -0.001624 xPosition (timeStamp) float64 88B -6.653e+06 -6.632e+06 ... -6.42e+06 yPosition (timeStamp) float64 88B 1.034e+06 1.043e+06 ... 1.126e+06 zPosition (timeStamp) float64 88B -2.479e+06 -2.532e+06 ... -3e+06 xVelocity (timeStamp) float64 88B 2.764e+03 2.82e+03 ... 3.316e+03 yVelocity (timeStamp) float64 88B 1.266e+03 1.254e+03 ... 1.143e+03 zVelocity (timeStamp) float64 88B -6.907e+03 -6.886e+03 ... -6.679e+03 Attributes: attitudeDataSource: Downlink attitudeOffsetsApplied: true Description: Attitude Information Data Store. Orbit Informati...
orbitAndAttitude- line: 11
- pixel: 11
- line(line)int640 1027 2055 ... 8220 9248 10276
- rasterAttributes_sampledLineSpacing_units :
- m
- rasterAttributes_sampledLineSpacing_value :
- 50.0
array([ 0, 1027, 2055, 3082, 4110, 5138, 6165, 7193, 8220, 9248, 10276])
- pixel(pixel)int640 1061 2123 ... 8493 9555 10617
- rasterAttributes_sampledPixelSpacing_units :
- m
- rasterAttributes_sampledPixelSpacing_value :
- 50.0
array([ 0, 1061, 2123, 3185, 4246, 5308, 6370, 7431, 8493, 9555, 10617])
- latitude(line, pixel)float64-19.82 -19.71 ... -23.23 -23.1
- units :
- deg
- xpath :
- /product/imageAttributes/geographicInformation/geolocationGrid/imageTiePoint/geodeticCoordinate/latitude
- Description :
- Geodetic latitude (units = deg)
array([[-19.82390748, -19.71020793, -19.59518856, -19.47886136, -19.36122117, -19.24226667, -19.1220062 , -19.00045628, -18.87763654, -18.75356264, -18.62823754], [-20.27630107, -20.16231142, -20.04696894, -19.93028583, -19.81225703, -19.69288137, -19.57216733, -19.45013163, -19.32679418, -19.20217088, -19.0762649 ], [-20.7286158 , -20.61433017, -20.49865901, -20.38161455, -20.26319178, -20.14338957, -20.02221652, -19.89968957, -19.77582886, -19.65065054, -19.52415799], [-21.18084653, -21.0662625 , -20.95025957, -20.83285024, -20.7140296 , -20.59379666, -20.4721602 , -20.34913737, -20.22474858, -20.09901026, -19.97192602], [-21.63299412, -21.51810544, -21.40176486, -21.28398491, -21.16476073, -21.04409139, -20.92198579, -20.79846131, -20.67353862, -20.5472344 , -20.41955247], [-22.08505314, -21.96985713, -21.85317556, -21.7350212 , -21.61538931, -21.4942791 , -21.37169966, -21.24766861, -21.12220688, -20.99533146, -20.86704641], [-22.53702463, -22.42151504, -22.3044865 , -22.18595187, -22.06590644, -21.94434951, -21.82129032, -21.69674671, -21.57073989, -21.44328712, -21.3143927 ], [-22.9889047 , -22.87307839, -22.75569914, -22.63678005, -22.51631656, -22.39430811, -22.27076412, -22.14570268, -22.01914531, -21.89110956, -21.76159999], [-23.44069154, -23.32454219, -23.20680616, -23.08749663, -22.96660909, -22.84414307, -22.72010818, -22.59452272, -22.4674085 , -22.33878338, -22.20865218], [-23.89238427, -23.77590827, -23.65781128, -23.53810674, -23.41679026, -23.29386151, -23.16933029, -23.04321519, -22.91553832, -22.78631785, -22.65555886], [-24.34397982, -24.22717071, -24.10870651, -23.98860074, -23.86684907, -23.74345129, -23.61841737, -23.49176614, -23.36352001, -23.23369749, -23.1023039 ]])
- longitude(line, pixel)float64168.8 168.3 167.8 ... 163.1 162.6
- units :
- deg
- xpath :
- /product/imageAttributes/geographicInformation/geolocationGrid/imageTiePoint/geodeticCoordinate/longitude
- Description :
- Geodetic longitude (units = deg)
array([[168.81472846, 168.32249998, 167.83104776, 167.3403784 , 166.85042686, 166.36114675, 165.8725324 , 165.38461077, 164.89741948, 164.41097839, 163.9252573 ], [168.70275313, 168.20919687, 167.71643791, 167.22448332, 166.7332681 , 166.24274597, 165.75291152, 165.26379215, 164.77542596, 164.28783332, 163.8009844 ], [168.59021038, 168.09528215, 167.60117481, 167.10789509, 166.61537758, 166.12357585, 165.63248458, 165.1421315 , 164.65255515, 164.16377635, 163.67576561], [168.4770878 , 167.98075825, 167.48527126, 166.9906341 , 166.49678145, 166.00366702, 165.51128579, 165.01966592, 164.52884651, 164.03884889, 163.54964396], [168.36337334, 167.86559656, 167.36868687, 166.87265114, 166.37742364, 165.8829579 , 165.38924904, 164.89632555, 164.404227 , 163.91297518, 163.42254136], [168.24903707, 167.7497828 , 167.25141823, 166.75395076, 166.25731472, 165.76146379, 165.26639337, 164.77213245, 164.27872114, 163.78618179, 163.29448607], [168.1340763 , 167.63329861, 167.13343584, 166.63449509, 166.13641032, 165.63913507, 165.14266489, 164.64702914, 164.15226843, 163.65840563, 163.16541277], [168.01846251, 167.51612903, 167.01473415, 166.51428544, 166.01471693, 165.51598225, 165.01807728, 164.52103186, 164.02488719, 163.52966671, 163.03534287], [167.90219443, 167.39825892, 166.89528815, 166.39328939, 165.89219631, 165.39196244, 164.89258381, 164.39409068, 163.89652478, 163.39991005, 162.90421938], [167.78524631, 167.27967433, 166.775092 , 166.27150701, 165.76885299, 165.26708358, 164.76619513, 164.26621839, 163.7671957 , 163.2691516 , 162.77205941], [167.66760391, 167.16034863, 166.65411027, 166.1488962 , 165.64463967, 165.1412942 , 164.63885631, 164.13735718, 163.6368397 , 163.13732896, 162.63879872]])
- height(line, pixel)float6419.01 19.01 19.01 ... 19.01 19.01
- units :
- m
- xpath :
- /product/imageAttributes/geographicInformation/geolocationGrid/imageTiePoint/geodeticCoordinate/height
- Description :
- Geodetic height above reference ellipsoid (units = m)
array([[19.00683975, 19.00683975, 19.00683975, 19.00683975, 19.00683975, 19.00683975, 19.00683975, 19.00683975, 19.00683975, 19.00683975, 19.00683975], [19.00683975, 19.00683975, 19.00683975, 19.00683975, 19.00683975, 19.00683975, 19.00683975, 19.00683975, 19.00683975, 19.00683975, 19.00683975], [19.00683975, 19.00683975, 19.00683975, 19.00683975, 19.00683975, 19.00683975, 19.00683975, 19.00683975, 19.00683975, 19.00683975, 19.00683975], [19.00683975, 19.00683975, 19.00683975, 19.00683975, 19.00683975, 19.00683975, 19.00683975, 19.00683975, 19.00683975, 19.00683975, 19.00683975], [19.00683975, 19.00683975, 19.00683975, 19.00683975, 19.00683975, 19.00683975, 19.00683975, 19.00683975, 19.00683975, 19.00683975, 19.00683975], [19.00683975, 19.00683975, 19.00683975, 19.00683975, 19.00683975, 19.00683975, 19.00683975, 19.00683975, 19.00683975, 19.00683975, 19.00683975], [19.00683975, 19.00683975, 19.00683975, 19.00683975, 19.00683975, 19.00683975, 19.00683975, 19.00683975, 19.00683975, 19.00683975, 19.00683975], [19.00683975, 19.00683975, 19.00683975, 19.00683975, 19.00683975, 19.00683975, 19.00683975, 19.00683975, 19.00683975, 19.00683975, 19.00683975], [19.00683975, 19.00683975, 19.00683975, 19.00683975, 19.00683975, 19.00683975, 19.00683975, 19.00683975, 19.00683975, 19.00683975, 19.00683975], [19.00683975, 19.00683975, 19.00683975, 19.00683975, 19.00683975, 19.00683975, 19.00683975, 19.00683975, 19.00683975, 19.00683975, 19.00683975], [19.00683975, 19.00683975, 19.00683975, 19.00683975, 19.00683975, 19.00683975, 19.00683975, 19.00683975, 19.00683975, 19.00683975, 19.00683975]])
- productFormat :
- GeoTIFF
- outputMediaInterleaving :
- BSQ
- rasterAttributes_dataType :
- Magnitude Detected
- rasterAttributes_bitsPerSample_dataStream :
- Magnitude
- rasterAttributes_bitsPerSample_value :
- 16
- rasterAttributes_numberOfSamplesPerLine :
- 10618
- rasterAttributes_numberOfLines :
- 10277
- rasterAttributes_sampledPixelSpacing_units :
- m
- rasterAttributes_sampledPixelSpacing_value :
- 50.0
- rasterAttributes_sampledLineSpacing_units :
- m
- rasterAttributes_sampledLineSpacing_value :
- 50.0
- rasterAttributes_lineTimeOrdering :
- Increasing
- rasterAttributes_pixelTimeOrdering :
- Decreasing
- footprint :
- POLYGON ((168.8147284622835 -19.82390747944243, 163.9252572974075 -18.62823753782336, 162.6387987182235 -23.10230390032916, 167.6676039112585 -24.34397982283393, 168.8147284622835 -19.82390747944243))
<xarray.DatasetView> Size: 3kB Dimensions: (line: 11, pixel: 11) Coordinates: * line (line) int64 88B 0 1027 2055 3082 4110 ... 7193 8220 9248 10276 * pixel (pixel) int64 88B 0 1061 2123 3185 4246 ... 7431 8493 9555 10617 Data variables: latitude (line, pixel) float64 968B -19.82 -19.71 -19.6 ... -23.23 -23.1 longitude (line, pixel) float64 968B 168.8 168.3 167.8 ... 163.1 162.6 height (line, pixel) float64 968B 19.01 19.01 19.01 ... 19.01 19.01 Attributes: (12/14) productFormat: GeoTIFF outputMediaInterleaving: BSQ rasterAttributes_dataType: Magnitude Detected rasterAttributes_bitsPerSample_dataStream: Magnitude rasterAttributes_bitsPerSample_value: 16 rasterAttributes_numberOfSamplesPerLine: 10618 ... ... rasterAttributes_sampledPixelSpacing_value: 50.0 rasterAttributes_sampledLineSpacing_units: m rasterAttributes_sampledLineSpacing_value: 50.0 rasterAttributes_lineTimeOrdering: Increasing rasterAttributes_pixelTimeOrdering: Decreasing footprint: POLYGON ((168.8147284622835 ...
geolocationGrid- timeOfDopplerCentroidEstimate: 6
- n-Coefficients: 5
- timeOfDopplerCentroidEstimate(timeOfDopplerCentroidEstimate)datetime64[ns]2022-04-07T18:21:27.475000 ... 2...
array(['2022-04-07T18:21:27.475000000', '2022-04-07T18:21:42.428868000', '2022-04-07T18:21:57.382736000', '2022-04-07T18:22:12.336603000', '2022-04-07T18:22:27.290472000', '2022-04-07T18:22:42.244341000'], dtype='datetime64[ns]')
- n-Coefficients(n-Coefficients)int640 1 2 3 4
array([0, 1, 2, 3, 4])
- dopplerAmbiguity(timeOfDopplerCentroidEstimate)int640 0 0 0 0 0
- xpath :
- /product/imageGenerationParameters/dopplerCentroid/dopplerAmbiguity
- Description :
- Doppler ambiguity number used during processing
array([0, 0, 0, 0, 0, 0])
- dopplerAmbiguityConfidence(timeOfDopplerCentroidEstimate)float640.9962 0.9962 ... 0.9962 0.9962
- xpath :
- /product/imageGenerationParameters/dopplerCentroid/dopplerAmbiguityConfidence
- Description :
- Doppler ambiguity confidence estimate.Range from 0.0 (no confidence) to 1.0 (highest confidence)
array([0.99622571, 0.99622571, 0.99622571, 0.99622571, 0.99622571, 0.99622571])
- dopplerCentroidReferenceTime(timeOfDopplerCentroidEstimate)float640.005613 0.005613 ... 0.005626
- units :
- s
- xpath :
- /product/imageGenerationParameters/dopplerCentroid/dopplerCentroidReferenceTime
- Description :
- 2-way slant range time used as reference in Doppler Centroid polynomial calculation (t0) (units = s)
array([0.00561258, 0.00561258, 0.00561258, 0.00561258, 0.00561258, 0.00562584])
- dopplerCentroidPolynomialPeriod(timeOfDopplerCentroidEstimate)float640.002049 0.002049 ... 0.002049
- units :
- s
- xpath :
- /product/imageGenerationParameters/dopplerCentroid/dopplerCentroidPolynomialPeriod
- Description :
- Approximate 2-way slant range time period in seconds corresponding to the slant range swath width. This is the period over which the Doppler centroid polynomial is valid, measured from Doppler centroid reference time (t0). For ScanSAR, this is the time corresponding to the full combined swath width.(units = s).
array([0.00204911, 0.00204911, 0.00204911, 0.00204911, 0.00204911, 0.00204911])
- dopplerCentroidCoefficients(timeOfDopplerCentroidEstimate, n-Coefficients)float64-196.1 7.52e+03 ... -2.112e+12
- xpath :
- /product/imageGenerationParameters/dopplerCentroid/dopplerCentroidCoefficients
- Description :
- List of up to 5 Doppler Centroid coefficients d0, d1, d2, d3, and d4 as a function of slant range timetSR where the Doppler Centroid frequency used in processing in Hz = d0 + d1(tSR - t0) + d2(tSR-t0)^2 + d3(tSR-t0)^3 + d4(tSR-t0)^4
array([[-1.96066406e+02, 7.52036621e+03, 7.81078700e+06, -5.08146022e+09, 1.86584098e+12], [-1.89800995e+02, 2.15785059e+04, -7.52412720e+07, 7.21335910e+10, -1.90014198e+13], [-2.14564896e+02, 1.66783188e+05, -1.88270304e+08, 3.63982111e+10, 1.26259298e+13], [-1.56520798e+02, -2.58028203e+05, 5.21606784e+08, -3.69894490e+11, 8.68939496e+13], [-1.99715393e+02, 1.50350266e+05, -1.87946704e+08, 8.88099717e+10, -1.39787302e+13], [-2.06129807e+02, 2.29637234e+05, -2.53270496e+08, 7.98278779e+10, -2.11173599e+12]])
- dopplerCentroidConfidence(timeOfDopplerCentroidEstimate)float640.9962 0.9977 ... 0.9948 0.9938
- xpath :
- /product/imageGenerationParameters/dopplerCentroid/dopplerCentroidConfidence
- Description :
- Doppler Centroid confidence estimate.Range from 0.0 (no confidence) to 1.0 (highest confidence)
array([0.99622571, 0.99767971, 0.99097651, 0.98627383, 0.99484169, 0.99383807])
- Description :
- Doppler Centroid Data Store
<xarray.DatasetView> Size: 568B Dimensions: (timeOfDopplerCentroidEstimate: 6, n-Coefficients: 5) Coordinates: * timeOfDopplerCentroidEstimate (timeOfDopplerCentroidEstimate) datetime64[ns] 48B ... * n-Coefficients (n-Coefficients) int64 40B 0 1 2 3 4 Data variables: dopplerAmbiguity (timeOfDopplerCentroidEstimate) int64 48B ... dopplerAmbiguityConfidence (timeOfDopplerCentroidEstimate) float64 48B ... dopplerCentroidReferenceTime (timeOfDopplerCentroidEstimate) float64 48B ... dopplerCentroidPolynomialPeriod (timeOfDopplerCentroidEstimate) float64 48B ... dopplerCentroidCoefficients (timeOfDopplerCentroidEstimate, n-Coefficients) float64 240B ... dopplerCentroidConfidence (timeOfDopplerCentroidEstimate) float64 48B ... Attributes: Description: Doppler Centroid Data Store
dopplerCentroid- dopplerRateReferenceTime: 1
- n-RateValuesCoefficients: 3
- dopplerRateReferenceTime(dopplerRateReferenceTime)float640.005613
array([0.005613])
- n-RateValuesCoefficients(n-RateValuesCoefficients)int640 1 2
array([0, 1, 2])
- dopplerRateValues(dopplerRateReferenceTime, n-RateValuesCoefficients)float64-2.162e+03 3.594e+05 -3.91e+07
- RateReferenceTime units :
- s
- dopplerRateReferenceTime_xpath :
- /product/imageGenerationParameters/dopplerRateValues/dopplerRateReferenceTime
- dopplerRateValuesCoefficients_xpath :
- /product/imageGenerationParameters/dopplerRateValues/dopplerRateValuesCoefficients
- Description :
- List of up to 5 Doppler rate values coefficients r0, r1, r2, r3, and r4 as a function of slant range time tSR where the Doppler frequency rate (in Hz per second of zero Doppler time) = r0 + r1(tSR - t0) + r2(tSR-t0)^2 + r3(tSR-t0)^3 + r4(tSR-t0)^4
array([[-2.16211890e+03, 3.59370219e+05, -3.90975000e+07]])
- Description :
- Doppler Rate Values Data Store.
<xarray.DatasetView> Size: 56B Dimensions: (dopplerRateReferenceTime: 1, n-RateValuesCoefficients: 3) Coordinates: * dopplerRateReferenceTime (dopplerRateReferenceTime) float64 8B 0.005613 * n-RateValuesCoefficients (n-RateValuesCoefficients) int64 24B 0 1 2 Data variables: dopplerRateValues (dopplerRateReferenceTime, n-RateValuesCoefficients) float64 24B ... Attributes: Description: Doppler Rate Values Data Store.
dopplerRateValues
<xarray.DatasetView> Size: 0B Dimensions: () Data variables: *empty*
doppler- pole: 2
- n-amplitudeCoefficients: 4
- n-phaseCoefficients: 4
- pole(pole)<U2'VV' 'VH'
- type :
- rs2prod:polarizationIdentifiers
array(['VV', 'VH'], dtype='<U2')
- n-amplitudeCoefficients(n-amplitudeCoefficients)int640 1 2 3
array([0, 1, 2, 3])
- n-phaseCoefficients(n-phaseCoefficients)int640 1 2 3
array([0, 1, 2, 3])
- replicaQualityValid(pole)<U4'true' 'true'
- xpath :
- /product/imageGenerationParameters/chirp/chirpQuality/replicaQualityValid
- Description :
- true = able to reconstruct all chirps or chirp reconstruction not requested (nominal chirp used) and all quality measures were acceptablefalse = unable to reconstruct chirp during processing and chirp reconstruction was requested or the quality is below acceptable levels.
array(['true', 'true'], dtype='<U4')
- crossCorrelationWidth(pole)float641.037 1.038
- xpath :
- /product/imageGenerationParameters/chirp/chirpQuality/crossCorrelationWidth
- Description :
- 3-dB pulse width of chirp replica cross-correlation function between reconstructed chirp and nominal chirp (units = samples)
array([1.03703594, 1.03756905])
- sideLobeLevel(pole)float64-13.33 -13.26
- xpath :
- /product/imageGenerationParameters/chirp/chirpQuality/sideLobeLevel
- units :
- dB
- Description :
- First side lobe level of chirp replica cross-correlation function between reconstructed chirp and nominal chirp (units = dB)
array([-13.32911968, -13.25835991])
- integratedSideLobeRatio(pole)float64-9.976 -10.16
- xpath :
- /product/imageGenerationParameters/chirp/chirpQuality/integratedSideLobeRatio
- units :
- dB
- Description :
- Integrated Side-Lobe Ratio of chirp replica cross-correlation function between reconstructed chirp and nominal chirp (units = dB)
array([ -9.97572708, -10.15859032])
- crossCorrelationPeakLoc(pole)float6438.61 38.21
- xpath :
- /product/imageGenerationParameters/chirp/chirpQuality/crossCorrelationPeakLoc
- Description :
- Cross correlation peak location. (units = samples)
array([38.60583496, 38.21051025])
- chirpPower(pole)float6464.28 64.17
- xpath :
- /product/imageGenerationParameters/chirp/chirpPower
- units :
- dB
- Description :
- (units = dB)
array([64.28054086, 64.16927446])
- amplitudeCoefficients(pole, n-amplitudeCoefficients)float641.0 -652.1 ... -2.642e+12
- xpath :
- /product/imageGenerationParameters/chirp/amplitudeCoefficients
- Description :
- List of range chirp amplitude coefficients (-, s^-1, s^-2, s^-3, ...)
array([[ 1.00000000e+00, -6.52071106e+02, 8.37702400e+06, -2.62762004e+12], [ 1.00000000e+00, -9.18793274e+02, -6.49115200e+07, -2.64203089e+12]])
- phaseCoefficients(pole, n-phaseCoefficients)float640.2689 1.349e+04 ... -1.012e+12
- xpath :
- /product/imageGenerationParameters/chirp/phaseCoefficients
- Description :
- List of range chirp phase coefficients (cycles, Hz, Hz/s, Hz/s^2, ...)
array([[ 2.68939286e-01, 1.34872969e+04, -1.39639505e+11, -5.13194787e+11], [ 8.32345009e-01, 4.97397266e+03, -1.39637899e+11, -1.01239901e+12]])
- VV_wing :
- Combined
- VV_pulse :
- 11.58
- VH_wing :
- Combined
- VH_pulse :
- 11.58
- Description :
- Chirp Data Store
<xarray.DatasetView> Size: 320B Dimensions: (pole: 2, n-amplitudeCoefficients: 4, n-phaseCoefficients: 4) Coordinates: * pole (pole) <U2 16B 'VV' 'VH' * n-amplitudeCoefficients (n-amplitudeCoefficients) int64 32B 0 1 2 3 * n-phaseCoefficients (n-phaseCoefficients) int64 32B 0 1 2 3 Data variables: replicaQualityValid (pole) <U4 32B 'true' 'true' crossCorrelationWidth (pole) float64 16B 1.037 1.038 sideLobeLevel (pole) float64 16B -13.33 -13.26 integratedSideLobeRatio (pole) float64 16B -9.976 -10.16 crossCorrelationPeakLoc (pole) float64 16B 38.61 38.21 chirpPower (pole) float64 16B 64.28 64.17 amplitudeCoefficients (pole, n-amplitudeCoefficients) float64 64B 1.0 ... phaseCoefficients (pole, n-phaseCoefficients) float64 64B 0.2689 .... Attributes: VV_wing: Combined VV_pulse: 11.58 VH_wing: Combined VH_pulse: 11.58 Description: Chirp Data Store
chirp
<xarray.DatasetView> Size: 0B Dimensions: () Data variables: *empty*
imageGenerationParameters- beam: 4
- pole: 2
- pixel: 99
- beam(beam)<U3'W1' 'W2' 'W30' 'S7'
array(['W1', 'W2', 'W30', 'S7'], dtype='<U3')
- pole(pole)<U2'VH' 'VV'
array(['VH', 'VV'], dtype='<U2')
- pixel(pixel)int640 1 2 3 4 5 6 ... 93 94 95 96 97 98
array([ 0, 1, 2, 3, 4, 5, 6, 7, 8, 9, 10, 11, 12, 13, 14, 15, 16, 17, 18, 19, 20, 21, 22, 23, 24, 25, 26, 27, 28, 29, 30, 31, 32, 33, 34, 35, 36, 37, 38, 39, 40, 41, 42, 43, 44, 45, 46, 47, 48, 49, 50, 51, 52, 53, 54, 55, 56, 57, 58, 59, 60, 61, 62, 63, 64, 65, 66, 67, 68, 69, 70, 71, 72, 73, 74, 75, 76, 77, 78, 79, 80, 81, 82, 83, 84, 85, 86, 87, 88, 89, 90, 91, 92, 93, 94, 95, 96, 97, 98])
- pulsesReceivedPerDwell(beam)int6458 58 58 58
- xpath :
- /product/sourceAttributes/radarParameters/pulsesReceivedPerDwell
- Description :
- ScanSAR burst parameter for ScanSAR only. Number of pulses received and recorded per dwell for each beam. Beam attribute values are same as those used in the beams list.
array([58, 58, 58, 58])
- numberOfPulseIntervalsPerDwell(beam)int6465 66 65 67
- xpath :
- /product/sourceAttributes/radarParameters/numberOfPulseIntervalsPerDwell
- Description :
- ScanSAR burst parameter for ScanSAR only. Number of pulse transmission intervals per dwell for each beam. Beam attribute values are same as those used in the beams list.
array([65, 66, 65, 67])
- rank(beam)int647 8 7 9
- xpath :
- /product/sourceAttributes/radarParameters/rank
- Description :
- Rank, one entry per beam. The number of transmitted pulse repetition intervals between transmission and reception for each beam. Samples per echo line. Used to determine sample window length. Beam attribute values are same as those used in the beams list.
array([7, 8, 7, 9])
- settableGain(beam, pole)float64-1.0 -1.0 -1.0 ... -1.0 -1.0 -1.0
- xpath :
- /product/sourceAttributes/radarParameters/settableGain
- wing :
- Combined
- units :
- dB
- Description :
- Gain values used on the instrument. Gain values will be specified either in terms of polarization or wing, not both. Beam attribute values are same as those used in the beams list. (units = dB). Wing can be either fore or aft.
array([[-1.00000002, -1.00000002], [-1.00000002, -1.00000002], [-1.00000002, -1.00000002], [-1.00000002, -1.00000002]])
- pulseRepetitionFrequency(beam)float641.272e+03 1.33e+03 ... 1.284e+03
- xpath :
- /product/sourceAttributes/radarParameters/pulseRepetitionFrequency
- units :
- Hz
- Description :
- Transmitted pulse repetition frequency for each beam. Beam attribute values are same as those used in the beams list (units = Hz). For products with two wing identifiers (Fore and Aft), the received PRF is effectively double the transmitted PRF.
array([1271.88439941, 1329.55175781, 1070.65319824, 1284.26281738])
- samplesPerEchoLine(beam)int646768 7920 7944 7320
- xpath :
- /product/sourceAttributes/radarParameters/samplesPerEchoLine
- Description :
- Number of samples per echo line for each beam. Used to determine sample window length. Beam attribute values are same as those used in the beams list.
array([6768, 7920, 7944, 7320])
- noiseLevelValues_BetaNought(pixel)float64-22.05 -23.83 ... -26.65 -25.52
- pixelFirstNoiseValue :
- 33
- stepSize :
- 108
- numberOfNoiseLevelValues :
- 99
- xpath :
- /product/sourceAttributes/radarParameters/referenceNoiseLevel/noiseLevelValues
- noiseLevelValues_units :
- dB
- Description :
- Estimated noise level values as a function of georeferenced image pixel position in range. For SSG and SPG products, these apply to intermediate georeferenced image data prior to geocoding. (units = dB)
array([-22.0480309, -23.8308601, -24.6454506, -24.8271008, -24.5917206, -24.2823792, -24.0880699, -24.16012 , -24.3325596, -24.4630699, -24.4747009, -24.3628807, -24.2323208, -24.1418209, -24.1742001, -24.3378296, -24.5296803, -24.6641808, -24.7199593, -24.7104893, -24.6347198, -24.5283108, -24.4534492, -24.5001793, -24.6506805, -24.8547897, -25.1622391, -25.4442997, -25.6879005, -25.6855793, -25.3954391, -24.6379108, -26.3336792, -26.6781902, -26.5182705, -26.0002708, -25.47616 , -24.9876404, -24.7475109, -24.9693298, -25.5615807, -26.2679996, -26.8668308, -27.1953201, -27.1978703, -26.8729706, -26.3505802, -25.7925892, -25.39958 , -25.4009094, -25.7947006, -26.49226 , -27.2579708, -27.8481808, -28.1557598, -28.0971909, -27.62146 , -26.7268295, -25.5062504, -25.5776691, -25.67976 , -25.8911991, -26.2002907, -26.52981 , -26.8151493, -26.9865208, -27.03685 , -26.9238396, -26.7098198, -26.4480991, -26.1808109, -26.0066204, -25.9750309, -26.0835495, -26.2642899, -26.4492493, -26.5925102, -26.5693398, -26.3428802, -28.0349197, -28.3677692, -28.3973598, -28.2351894, -27.9366703, -27.5906696, -27.2849808, -27.0888195, -27.0662594, -27.2208996, -27.5067596, -27.86273 , -28.2094593, -28.4701309, -28.5607796, -28.4260101, -28.05089 , -27.4847393, -26.6474609, -25.5237503])
- noiseLevelValues_SigmaNought(pixel)float64-26.78 -28.48 ... -27.84 -26.7
- pixelFirstNoiseValue :
- 33
- stepSize :
- 108
- numberOfNoiseLevelValues :
- 99
- xpath :
- /product/sourceAttributes/radarParameters/referenceNoiseLevel/noiseLevelValues
- noiseLevelValues_units :
- dB
- Description :
- Estimated noise level values as a function of georeferenced image pixel position in range. For SSG and SPG products, these apply to intermediate georeferenced image data prior to geocoding. (units = dB)
array([-26.7759094, -28.4762707, -29.2104492, -29.3136806, -29.0017891, -28.6177807, -28.3505707, -28.3514309, -28.4543304, -28.5168991, -28.4621296, -28.2853909, -28.0913601, -27.9387798, -27.9104195, -28.0146294, -28.1483307, -28.2259007, -28.2259407, -28.1618996, -28.0326595, -27.8738995, -27.7477303, -27.7441807, -27.8454094, -28.0012207, -28.2613201, -28.4969406, -28.6950207, -28.6480408, -28.3140793, -27.5135803, -29.1671791, -29.4703102, -29.2697906, -28.7119293, -28.1486893, -27.6217594, -27.3439102, -27.5286903, -28.0845699, -28.75527 , -29.3190193, -29.6130295, -29.5817204, -29.2235508, -28.6684608, -28.0783501, -27.6537609, -27.6240501, -27.9873409, -28.6548996, -29.39114 , -29.9523506, -30.2314301, -30.1448402, -29.6415405, -28.7198105, -27.4725704, -27.5177708, -27.5940495, -27.7801208, -28.0642395, -28.3691998, -28.6303596, -28.7779503, -28.8048706, -28.6688194, -28.4321308, -28.1480999, -27.8588409, -27.6630306, -27.6101398, -27.69771 , -27.8578091, -28.0224609, -28.14571 , -28.1028309, -27.8569794, -29.5299091, -29.8439407, -29.8549995, -29.6745605, -29.3580608, -28.9943409, -28.6711998, -28.45784 , -28.4183407, -28.5562801, -28.8257008, -29.1654491, -29.4962006, -29.7411308, -29.8162594, -29.6662006, -29.2759991, -28.6949902, -27.84305 , -26.7049007])
- noiseLevelValues_Gamma(pixel)float64-26.51 -28.2 ... -25.98 -24.82
- pixelFirstNoiseValue :
- 33
- stepSize :
- 108
- numberOfNoiseLevelValues :
- 99
- xpath :
- /product/sourceAttributes/radarParameters/referenceNoiseLevel/noiseLevelValues
- noiseLevelValues_units :
- dB
- Description :
- Estimated noise level values as a function of georeferenced image pixel position in range. For SSG and SPG products, these apply to intermediate georeferenced image data prior to geocoding. (units = dB)
array([-26.5146694, -28.2042599, -28.9274807, -29.0195599, -28.6963501, -28.3008308, -28.0219402, -28.0109501, -28.10182 , -28.1521893, -28.0850506, -27.8957806, -27.6890507, -27.5236206, -27.4822502, -27.5732803, -27.6936607, -27.7577496, -27.7441597, -27.6663494, -27.5231991, -27.3503704, -27.2099991, -27.1921101, -27.2788696, -27.4200706, -27.6654301, -27.8861809, -28.0692596, -28.0071602, -27.6579609, -26.8420906, -28.4802094, -28.7677402, -28.5514908, -27.9778099, -27.3986301, -26.8556404, -26.5616398, -26.7301693, -27.26968 , -27.9239101, -28.4710999, -28.7484493, -28.7003899, -28.3253593, -27.7533302, -27.1461906, -26.7044792, -26.6575699, -27.0035591, -27.6537495, -28.37253 , -28.9162102, -29.1776695, -29.0733891, -28.55233 , -27.6127491, -26.3476009, -26.3748093, -26.4330502, -26.6009998, -26.86693 , -27.1536293, -27.3964806, -27.5256901, -27.5341702, -27.3796291, -27.1243801, -26.8217392, -26.5138092, -26.2992802, -26.2276192, -26.2963505, -26.4375801, -26.5832901, -26.6875706, -26.6256695, -26.3607407, -28.0145607, -28.3094196, -28.3012695, -28.1015892, -27.7658005, -27.38274 , -27.0402298, -26.8074608, -26.7485104, -26.8669605, -27.1168499, -27.4370499, -27.7481995, -27.9734993, -28.0289593, -27.8591995, -27.4492702, -26.8484993, -25.9767704, -24.8187904])
- acquisitionType :
- ScanSAR Wide
- beams :
- ['W1', 'W2', 'W30', 'S7']
- polarizations :
- ['VV', 'VH']
- pulses :
- 11.58
- radarCenterFrequency_units :
- Hz
- radarCenterFrequency :
- 5404999242.769673
- pulseLength_pulse :
- 11.58
- pulseLength_units :
- s
- pulseLength :
- 4.152204895019531e-05
- pulseBandwidth_pulse :
- 11.58
- pulseBandwidth_units :
- Hz
- pulseBandwidth :
- 11597107.86
- antennaPointing :
- Right
- adcSamplingRate_pulse :
- 11.58
- adcSamplingRate_units :
- Hz
- adcSamplingRate :
- 12667968.75
- yawSteeringFlag :
- YawSteeringOn
- geodeticFlag :
- Off-Geocentric
- rawBitsPerSample :
- 4
- Description :
- Radar Parameters Data Store. Information describing the characteristics of the sensor used to acquire the data
<xarray.DatasetView> Size: 3kB Dimensions: (beam: 4, pole: 2, pixel: 99) Coordinates: * beam (beam) <U3 48B 'W1' 'W2' 'W30' 'S7' * pole (pole) <U2 16B 'VH' 'VV' * pixel (pixel) int64 792B 0 1 2 3 4 ... 95 96 97 98 Data variables: pulsesReceivedPerDwell (beam) int64 32B 58 58 58 58 numberOfPulseIntervalsPerDwell (beam) int64 32B 65 66 65 67 rank (beam) int64 32B 7 8 7 9 settableGain (beam, pole) float64 64B -1.0 -1.0 ... -1.0 pulseRepetitionFrequency (beam) float64 32B 1.272e+03 ... 1.284e+03 samplesPerEchoLine (beam) int64 32B 6768 7920 7944 7320 noiseLevelValues_BetaNought (pixel) float64 792B -22.05 ... -25.52 noiseLevelValues_SigmaNought (pixel) float64 792B -26.78 -28.48 ... -26.7 noiseLevelValues_Gamma (pixel) float64 792B -26.51 -28.2 ... -24.82 Attributes: (12/20) acquisitionType: ScanSAR Wide beams: ['W1', 'W2', 'W30', 'S7'] polarizations: ['VV', 'VH'] pulses: 11.58 radarCenterFrequency_units: Hz radarCenterFrequency: 5404999242.769673 ... ... adcSamplingRate_units: Hz adcSamplingRate: 12667968.75 yawSteeringFlag: YawSteeringOn geodeticFlag: Off-Geocentric rawBitsPerSample: 4 Description: Radar Parameters Data Store. Information des...
radarParameters- pixel: 10618
- pixel(pixel)int640 1 2 3 ... 10614 10615 10616 10617
array([ 0, 1, 2, ..., 10615, 10616, 10617])
- lutSigma(pixel)float644.071e+07 4.07e+07 ... 1.785e+07
- offset :
- 0.0
array([40709550., 40702230., 40694940., ..., 17846740., 17846190., 17845640.])
- lutGamma(pixel)float643.838e+07 3.837e+07 ... 1.157e+07
- offset :
- 0.0
array([38376630., 38368870., 38361140., ..., 11576030., 11575180., 11574340.])
- lutBeta(pixel)float641.358e+07 1.358e+07 ... 1.358e+07
- offset :
- 0.0
array([13583140., 13583140., 13583140., ..., 13583140., 13583140., 13583140.])
- Description :
- RADARSAT Product LUT. (c) COPYRIGHT MacDonald Dettwiler and Associates Ltd., 2003 All Rights Reserved.Three output scaling Look-up Tables (LUTs) are included with every product. These LUTs allow one to convert the digital numbers foundin the output product to sigma-nought, beta-nought, or gamma-noughtvalues (depending on which LUT is used) by applying a constantoffset and range dependent gain to the SAR imagery.There is one entry in the gains list for each range sample in theimagery. In order to convert the digital number of a given rangesample to a calibrated value, the digital value is first squared,then the offset (B) is added and the result is divided by thegain value (A) corresponding to the range sample.i.e., calibrated value = ( digital value^2 + B ) / A
<xarray.DatasetView> Size: 340kB Dimensions: (pixel: 10618) Coordinates: * pixel (pixel) int64 85kB 0 1 2 3 4 5 ... 10613 10614 10615 10616 10617 Data variables: lutSigma (pixel) float64 85kB 4.071e+07 4.07e+07 ... 1.785e+07 1.785e+07 lutGamma (pixel) float64 85kB 3.838e+07 3.837e+07 ... 1.158e+07 1.157e+07 lutBeta (pixel) float64 85kB 1.358e+07 1.358e+07 ... 1.358e+07 1.358e+07 Attributes: Description: RADARSAT Product LUT. (c) COPYRIGHT MacDonald Dettwiler and...
lut- product_path :
- /home1/scratch/agrouaze/xsardatasync/xsardata/RS2_OK135107_PK1187782_DK1151894_SCWA_20220407_182127_VV_VH_SGF
- satellite :
- RADARSAT-2
- inputDatasetId :
- /Fred/RSAT-2/610044P
- rawDataStartTime :
- 2022-04-07T18:21:27.688416000
- satelliteHeight_units :
- m
- satelliteHeight :
- 800612.0083192665
- passDirection :
- Descending
Examples of alias to datasets (from the datatree above)
[4]:
#geolocation grid (low resolution)
rs2meta.geoloc
[4]:
<xarray.Dataset> Size: 3kB Dimensions: (line: 11, pixel: 11) Coordinates: * line (line) int64 88B 0 1027 2055 3082 4110 ... 7193 8220 9248 10276 * pixel (pixel) int64 88B 0 1061 2123 3185 4246 ... 7431 8493 9555 10617 Data variables: latitude (line, pixel) float64 968B -19.82 -19.71 -19.6 ... -23.23 -23.1 longitude (line, pixel) float64 968B 168.8 168.3 167.8 ... 163.1 162.6 height (line, pixel) float64 968B 19.01 19.01 19.01 ... 19.01 19.01 Attributes: (12/14) productFormat: GeoTIFF outputMediaInterleaving: BSQ rasterAttributes_dataType: Magnitude Detected rasterAttributes_bitsPerSample_dataStream: Magnitude rasterAttributes_bitsPerSample_value: 16 rasterAttributes_numberOfSamplesPerLine: 10618 ... ... rasterAttributes_sampledPixelSpacing_value: 50.0 rasterAttributes_sampledLineSpacing_units: m rasterAttributes_sampledLineSpacing_value: 50.0 rasterAttributes_lineTimeOrdering: Increasing rasterAttributes_pixelTimeOrdering: Decreasing footprint: POLYGON ((168.8147284622835 ...
- line: 11
- pixel: 11
- line(line)int640 1027 2055 ... 8220 9248 10276
- rasterAttributes_sampledLineSpacing_units :
- m
- rasterAttributes_sampledLineSpacing_value :
- 50.0
array([ 0, 1027, 2055, 3082, 4110, 5138, 6165, 7193, 8220, 9248, 10276])
- pixel(pixel)int640 1061 2123 ... 8493 9555 10617
- rasterAttributes_sampledPixelSpacing_units :
- m
- rasterAttributes_sampledPixelSpacing_value :
- 50.0
array([ 0, 1061, 2123, 3185, 4246, 5308, 6370, 7431, 8493, 9555, 10617])
- latitude(line, pixel)float64-19.82 -19.71 ... -23.23 -23.1
- units :
- deg
- xpath :
- /product/imageAttributes/geographicInformation/geolocationGrid/imageTiePoint/geodeticCoordinate/latitude
- Description :
- Geodetic latitude (units = deg)
array([[-19.82390748, -19.71020793, -19.59518856, -19.47886136, -19.36122117, -19.24226667, -19.1220062 , -19.00045628, -18.87763654, -18.75356264, -18.62823754], [-20.27630107, -20.16231142, -20.04696894, -19.93028583, -19.81225703, -19.69288137, -19.57216733, -19.45013163, -19.32679418, -19.20217088, -19.0762649 ], [-20.7286158 , -20.61433017, -20.49865901, -20.38161455, -20.26319178, -20.14338957, -20.02221652, -19.89968957, -19.77582886, -19.65065054, -19.52415799], [-21.18084653, -21.0662625 , -20.95025957, -20.83285024, -20.7140296 , -20.59379666, -20.4721602 , -20.34913737, -20.22474858, -20.09901026, -19.97192602], [-21.63299412, -21.51810544, -21.40176486, -21.28398491, -21.16476073, -21.04409139, -20.92198579, -20.79846131, -20.67353862, -20.5472344 , -20.41955247], [-22.08505314, -21.96985713, -21.85317556, -21.7350212 , -21.61538931, -21.4942791 , -21.37169966, -21.24766861, -21.12220688, -20.99533146, -20.86704641], [-22.53702463, -22.42151504, -22.3044865 , -22.18595187, -22.06590644, -21.94434951, -21.82129032, -21.69674671, -21.57073989, -21.44328712, -21.3143927 ], [-22.9889047 , -22.87307839, -22.75569914, -22.63678005, -22.51631656, -22.39430811, -22.27076412, -22.14570268, -22.01914531, -21.89110956, -21.76159999], [-23.44069154, -23.32454219, -23.20680616, -23.08749663, -22.96660909, -22.84414307, -22.72010818, -22.59452272, -22.4674085 , -22.33878338, -22.20865218], [-23.89238427, -23.77590827, -23.65781128, -23.53810674, -23.41679026, -23.29386151, -23.16933029, -23.04321519, -22.91553832, -22.78631785, -22.65555886], [-24.34397982, -24.22717071, -24.10870651, -23.98860074, -23.86684907, -23.74345129, -23.61841737, -23.49176614, -23.36352001, -23.23369749, -23.1023039 ]])
- longitude(line, pixel)float64168.8 168.3 167.8 ... 163.1 162.6
- units :
- deg
- xpath :
- /product/imageAttributes/geographicInformation/geolocationGrid/imageTiePoint/geodeticCoordinate/longitude
- Description :
- Geodetic longitude (units = deg)
array([[168.81472846, 168.32249998, 167.83104776, 167.3403784 , 166.85042686, 166.36114675, 165.8725324 , 165.38461077, 164.89741948, 164.41097839, 163.9252573 ], [168.70275313, 168.20919687, 167.71643791, 167.22448332, 166.7332681 , 166.24274597, 165.75291152, 165.26379215, 164.77542596, 164.28783332, 163.8009844 ], [168.59021038, 168.09528215, 167.60117481, 167.10789509, 166.61537758, 166.12357585, 165.63248458, 165.1421315 , 164.65255515, 164.16377635, 163.67576561], [168.4770878 , 167.98075825, 167.48527126, 166.9906341 , 166.49678145, 166.00366702, 165.51128579, 165.01966592, 164.52884651, 164.03884889, 163.54964396], [168.36337334, 167.86559656, 167.36868687, 166.87265114, 166.37742364, 165.8829579 , 165.38924904, 164.89632555, 164.404227 , 163.91297518, 163.42254136], [168.24903707, 167.7497828 , 167.25141823, 166.75395076, 166.25731472, 165.76146379, 165.26639337, 164.77213245, 164.27872114, 163.78618179, 163.29448607], [168.1340763 , 167.63329861, 167.13343584, 166.63449509, 166.13641032, 165.63913507, 165.14266489, 164.64702914, 164.15226843, 163.65840563, 163.16541277], [168.01846251, 167.51612903, 167.01473415, 166.51428544, 166.01471693, 165.51598225, 165.01807728, 164.52103186, 164.02488719, 163.52966671, 163.03534287], [167.90219443, 167.39825892, 166.89528815, 166.39328939, 165.89219631, 165.39196244, 164.89258381, 164.39409068, 163.89652478, 163.39991005, 162.90421938], [167.78524631, 167.27967433, 166.775092 , 166.27150701, 165.76885299, 165.26708358, 164.76619513, 164.26621839, 163.7671957 , 163.2691516 , 162.77205941], [167.66760391, 167.16034863, 166.65411027, 166.1488962 , 165.64463967, 165.1412942 , 164.63885631, 164.13735718, 163.6368397 , 163.13732896, 162.63879872]])
- height(line, pixel)float6419.01 19.01 19.01 ... 19.01 19.01
- units :
- m
- xpath :
- /product/imageAttributes/geographicInformation/geolocationGrid/imageTiePoint/geodeticCoordinate/height
- Description :
- Geodetic height above reference ellipsoid (units = m)
array([[19.00683975, 19.00683975, 19.00683975, 19.00683975, 19.00683975, 19.00683975, 19.00683975, 19.00683975, 19.00683975, 19.00683975, 19.00683975], [19.00683975, 19.00683975, 19.00683975, 19.00683975, 19.00683975, 19.00683975, 19.00683975, 19.00683975, 19.00683975, 19.00683975, 19.00683975], [19.00683975, 19.00683975, 19.00683975, 19.00683975, 19.00683975, 19.00683975, 19.00683975, 19.00683975, 19.00683975, 19.00683975, 19.00683975], [19.00683975, 19.00683975, 19.00683975, 19.00683975, 19.00683975, 19.00683975, 19.00683975, 19.00683975, 19.00683975, 19.00683975, 19.00683975], [19.00683975, 19.00683975, 19.00683975, 19.00683975, 19.00683975, 19.00683975, 19.00683975, 19.00683975, 19.00683975, 19.00683975, 19.00683975], [19.00683975, 19.00683975, 19.00683975, 19.00683975, 19.00683975, 19.00683975, 19.00683975, 19.00683975, 19.00683975, 19.00683975, 19.00683975], [19.00683975, 19.00683975, 19.00683975, 19.00683975, 19.00683975, 19.00683975, 19.00683975, 19.00683975, 19.00683975, 19.00683975, 19.00683975], [19.00683975, 19.00683975, 19.00683975, 19.00683975, 19.00683975, 19.00683975, 19.00683975, 19.00683975, 19.00683975, 19.00683975, 19.00683975], [19.00683975, 19.00683975, 19.00683975, 19.00683975, 19.00683975, 19.00683975, 19.00683975, 19.00683975, 19.00683975, 19.00683975, 19.00683975], [19.00683975, 19.00683975, 19.00683975, 19.00683975, 19.00683975, 19.00683975, 19.00683975, 19.00683975, 19.00683975, 19.00683975, 19.00683975], [19.00683975, 19.00683975, 19.00683975, 19.00683975, 19.00683975, 19.00683975, 19.00683975, 19.00683975, 19.00683975, 19.00683975, 19.00683975]])
- linePandasIndex
PandasIndex(Index([0, 1027, 2055, 3082, 4110, 5138, 6165, 7193, 8220, 9248, 10276], dtype='int64', name='line'))
- pixelPandasIndex
PandasIndex(Index([0, 1061, 2123, 3185, 4246, 5308, 6370, 7431, 8493, 9555, 10617], dtype='int64', name='pixel'))
- productFormat :
- GeoTIFF
- outputMediaInterleaving :
- BSQ
- rasterAttributes_dataType :
- Magnitude Detected
- rasterAttributes_bitsPerSample_dataStream :
- Magnitude
- rasterAttributes_bitsPerSample_value :
- 16
- rasterAttributes_numberOfSamplesPerLine :
- 10618
- rasterAttributes_numberOfLines :
- 10277
- rasterAttributes_sampledPixelSpacing_units :
- m
- rasterAttributes_sampledPixelSpacing_value :
- 50.0
- rasterAttributes_sampledLineSpacing_units :
- m
- rasterAttributes_sampledLineSpacing_value :
- 50.0
- rasterAttributes_lineTimeOrdering :
- Increasing
- rasterAttributes_pixelTimeOrdering :
- Decreasing
- footprint :
- POLYGON ((168.8147284622835 -19.82390747944243, 163.9252572974075 -18.62823753782336, 162.6387987182235 -23.10230390032916, 167.6676039112585 -24.34397982283393, 168.8147284622835 -19.82390747944243))
[5]:
#Calibration look up tables in range
rs2meta.lut
[5]:
<xarray.DatasetView> Size: 340kB Dimensions: (pixel: 10618) Coordinates: * pixel (pixel) int64 85kB 0 1 2 3 4 5 ... 10613 10614 10615 10616 10617 Data variables: lutSigma (pixel) float64 85kB 4.071e+07 4.07e+07 ... 1.785e+07 1.785e+07 lutGamma (pixel) float64 85kB 3.838e+07 3.837e+07 ... 1.158e+07 1.157e+07 lutBeta (pixel) float64 85kB 1.358e+07 1.358e+07 ... 1.358e+07 1.358e+07 Attributes: Description: RADARSAT Product LUT. (c) COPYRIGHT MacDonald Dettwiler and...
- pixel: 10618
- pixel(pixel)int640 1 2 3 ... 10614 10615 10616 10617
array([ 0, 1, 2, ..., 10615, 10616, 10617])
- lutSigma(pixel)float644.071e+07 4.07e+07 ... 1.785e+07
- offset :
- 0.0
array([40709550., 40702230., 40694940., ..., 17846740., 17846190., 17845640.])
- lutGamma(pixel)float643.838e+07 3.837e+07 ... 1.157e+07
- offset :
- 0.0
array([38376630., 38368870., 38361140., ..., 11576030., 11575180., 11574340.])
- lutBeta(pixel)float641.358e+07 1.358e+07 ... 1.358e+07
- offset :
- 0.0
array([13583140., 13583140., 13583140., ..., 13583140., 13583140., 13583140.])
- pixelPandasIndex
PandasIndex(Index([ 0, 1, 2, 3, 4, 5, 6, 7, 8, 9, ... 10608, 10609, 10610, 10611, 10612, 10613, 10614, 10615, 10616, 10617], dtype='int64', name='pixel', length=10618))
- Description :
- RADARSAT Product LUT. (c) COPYRIGHT MacDonald Dettwiler and Associates Ltd., 2003 All Rights Reserved.Three output scaling Look-up Tables (LUTs) are included with every product. These LUTs allow one to convert the digital numbers foundin the output product to sigma-nought, beta-nought, or gamma-noughtvalues (depending on which LUT is used) by applying a constantoffset and range dependent gain to the SAR imagery.There is one entry in the gains list for each range sample in theimagery. In order to convert the digital number of a given rangesample to a calibrated value, the digital value is first squared,then the offset (B) is added and the result is divided by thegain value (A) corresponding to the range sample.i.e., calibrated value = ( digital value^2 + B ) / A
Open a dataset
[6]:
# Define the resolution to load the dataset at a lower resolution (if not specified or None, the dataset is loaded at high resolution)
resolution = '1000m'
# Instanciate a RadarSatDataset object
rs2ds = xsar.RadarSat2Dataset(dataset_id=path, resolution=resolution)
/home/datawork-cersat-public/cache/project/mpc-sentinel1/workspace/mamba/envs/testgeoviewsnumpy/lib/python3.11/site-packages/xradarsat2/radarSat2_xarray_reader.py:299: UserWarning: no explicit representation of timezones available for np.datetime64
timestamp.append(np.datetime64(value["timeStamp"]).astype("datetime64[ns]"))
/home/datawork-cersat-public/cache/project/mpc-sentinel1/workspace/mamba/envs/testgeoviewsnumpy/lib/python3.11/site-packages/xradarsat2/radarSat2_xarray_reader.py:462: UserWarning: no explicit representation of timezones available for np.datetime64
timestamp.append(np.datetime64(value["timeStamp"]).astype("datetime64[ns]"))
/home/datawork-cersat-public/cache/project/mpc-sentinel1/workspace/mamba/envs/testgeoviewsnumpy/lib/python3.11/site-packages/xradarsat2/radarSat2_xarray_reader.py:570: UserWarning: no explicit representation of timezones available for np.datetime64
times.append(np.datetime64(value["timeOfDopplerCentroidEstimate"]).astype("datetime64[ns]"))
/home/datawork-cersat-public/cache/project/mpc-sentinel1/workspace/mamba/envs/testgeoviewsnumpy/lib/python3.11/site-packages/xradarsat2/radarSat2_xarray_reader.py:2056: UserWarning: no explicit representation of timezones available for np.datetime64
final_dic[key] = np.datetime64(dic[key]).astype("datetime64[ns]")
Get the Dataset object
[7]:
rs2ds
[7]:
<RadarSat2Dataset full coverage object>
Access the metadata object from the Dataset object
[8]:
rs2ds.sar_meta
[8]:
<BlockingActorProxy: RadarSat2Meta>
Access the dataset
In this dataset, we can find variables like latitude, longitude, look up tables (before and after denoising), incidence…
[9]:
rs2ds.dataset
[9]:
<xarray.Dataset> Size: 46MB Dimensions: (line: 513, pol: 2, sample: 530) Coordinates: * line (line) float64 4kB 9.5 29.5 49.5 ... 1.023e+04 1.025e+04 * pol (pol) <U2 16B 'VV' 'VH' * sample (sample) float64 4kB 9.5 29.5 49.5 ... 1.057e+04 1.059e+04 Data variables: (12/23) digital_number (pol, line, sample) uint16 1MB dask.array<chunksize=(1, 513, 530), meta=np.ndarray> lines_flipped bool 1B False samples_flipped bool 1B True sampleSpacing float64 8B 1e+03 lineSpacing float64 8B 1e+03 sigma0_raw (pol, line, sample) float64 4MB dask.array<chunksize=(1, 513, 530), meta=np.ndarray> ... ... time (line) datetime64[ns] 4kB dask.array<chunksize=(513,), meta=np.ndarray> latitude (line, sample) float64 2MB dask.array<chunksize=(513, 530), meta=np.ndarray> longitude (line, sample) float64 2MB dask.array<chunksize=(513, 530), meta=np.ndarray> altitude (line, sample) float64 2MB dask.array<chunksize=(513, 530), meta=np.ndarray> incidence (line, sample) float64 2MB dask.array<chunksize=(513, 530), meta=np.ndarray> elevation (line, sample) float64 2MB dask.array<chunksize=(513, 530), meta=np.ndarray> Attributes: (12/18) product_path: /home1/scratch/agrouaze/xsardatasync/xsardata/RS2... satellite: RADARSAT-2 inputDatasetId: /Fred/RSAT-2/610044P rawDataStartTime: 2022-04-07T18:21:27.688416000 satelliteHeight_units: m satelliteHeight: 800612.0083192665 ... ... stop_date: 2022-04-07 18:22:44.030964 footprint: POLYGON ((168.8147284622835 -19.82390747944243, 1... coverage: 515km * 530km (line * sample ) pixel_line_m: 50.0 pixel_sample_m: 50.0 approx_transform: |-0.00,-0.00, 168.85|\n|-0.00, 0.00,-19.85|\n| 0....
- line: 513
- pol: 2
- sample: 530
- line(line)float649.5 29.5 ... 1.023e+04 1.025e+04
array([9.50000e+00, 2.95000e+01, 4.95000e+01, ..., 1.02095e+04, 1.02295e+04, 1.02495e+04])
- pol(pol)<U2'VV' 'VH'
array(['VV', 'VH'], dtype='<U2')
- sample(sample)float649.5 29.5 ... 1.057e+04 1.059e+04
array([9.50000e+00, 2.95000e+01, 4.95000e+01, ..., 1.05495e+04, 1.05695e+04, 1.05895e+04])
- digital_number(pol, line, sample)uint16dask.array<chunksize=(1, 513, 530), meta=np.ndarray>
- comment :
- not denoised digital number, resampled at "1000m" with rasterio.enums.Resampling.rms
- history :
- digital_number: imagery_V*.tif
Array Chunk Bytes 1.04 MiB 531.04 kiB Shape (2, 513, 530) (1, 513, 530) Dask graph 2 chunks in 4 graph layers Data type uint16 numpy.ndarray - lines_flipped()boolFalse
- meaning :
- xsar convention : increasing time along line axis (whatever ascending or descending pass direction)
array(False)
- samples_flipped()boolTrue
- meaning :
- xsar convention : increasing incidence values along samples axis
array(True)
- sampleSpacing()float641e+03
- units :
- m
- referential :
- ground
array(1000.)
- lineSpacing()float641e+03
- units :
- m
array(1000.)
- sigma0_raw(pol, line, sample)float64dask.array<chunksize=(1, 513, 530), meta=np.ndarray>
Array Chunk Bytes 4.15 MiB 2.07 MiB Shape (2, 513, 530) (1, 513, 530) Dask graph 2 chunks in 20 graph layers Data type float64 numpy.ndarray - nesz(pol, line, sample)float32dask.array<chunksize=(1, 513, 530), meta=np.ndarray>
Array Chunk Bytes 2.07 MiB 1.04 MiB Shape (2, 513, 530) (1, 513, 530) Dask graph 2 chunks in 11 graph layers Data type float32 numpy.ndarray - gamma0_raw(pol, line, sample)float64dask.array<chunksize=(1, 513, 530), meta=np.ndarray>
Array Chunk Bytes 4.15 MiB 2.07 MiB Shape (2, 513, 530) (1, 513, 530) Dask graph 2 chunks in 20 graph layers Data type float64 numpy.ndarray - negz(pol, line, sample)float32dask.array<chunksize=(1, 513, 530), meta=np.ndarray>
Array Chunk Bytes 2.07 MiB 1.04 MiB Shape (2, 513, 530) (1, 513, 530) Dask graph 2 chunks in 11 graph layers Data type float32 numpy.ndarray - beta0_raw(pol, line, sample)float64dask.array<chunksize=(1, 513, 530), meta=np.ndarray>
Array Chunk Bytes 4.15 MiB 2.07 MiB Shape (2, 513, 530) (1, 513, 530) Dask graph 2 chunks in 20 graph layers Data type float64 numpy.ndarray - nebz(pol, line, sample)float32dask.array<chunksize=(1, 513, 530), meta=np.ndarray>
Array Chunk Bytes 2.07 MiB 1.04 MiB Shape (2, 513, 530) (1, 513, 530) Dask graph 2 chunks in 11 graph layers Data type float32 numpy.ndarray - sigma0(pol, line, sample)float64dask.array<chunksize=(1, 513, 530), meta=np.ndarray>
- comment :
- not clipped, some values can be <0
Array Chunk Bytes 4.15 MiB 2.07 MiB Shape (2, 513, 530) (1, 513, 530) Dask graph 2 chunks in 31 graph layers Data type float64 numpy.ndarray - beta0(pol, line, sample)float64dask.array<chunksize=(1, 513, 530), meta=np.ndarray>
- comment :
- not clipped, some values can be <0
Array Chunk Bytes 4.15 MiB 2.07 MiB Shape (2, 513, 530) (1, 513, 530) Dask graph 2 chunks in 31 graph layers Data type float64 numpy.ndarray - gamma0(pol, line, sample)float64dask.array<chunksize=(1, 513, 530), meta=np.ndarray>
- comment :
- not clipped, some values can be <0
Array Chunk Bytes 4.15 MiB 2.07 MiB Shape (2, 513, 530) (1, 513, 530) Dask graph 2 chunks in 31 graph layers Data type float64 numpy.ndarray - land_mask(line, sample)int8dask.array<chunksize=(513, 530), meta=np.ndarray>
- history :
- land_mask: cartopy.feature.NaturalEarthFeature land
- meaning :
- 0: ocean , 1: land
Array Chunk Bytes 265.52 kiB 265.52 kiB Shape (513, 530) (513, 530) Dask graph 1 chunks in 3 graph layers Data type int8 numpy.ndarray - velocity(line)float647.546e+03 7.546e+03 ... 7.544e+03
array([7546.25416882, 7546.25047109, 7546.24677337, 7546.24307564, 7546.23937792, 7546.2356802 , 7546.23198248, 7546.22828475, 7546.22458703, 7546.22088931, 7546.21719158, 7546.21349386, 7546.20979613, 7546.20609842, 7546.20240069, 7546.19870297, 7546.19500524, 7546.19130752, 7546.18760979, 7546.18391207, 7546.18021435, 7546.17651663, 7546.1728189 , 7546.16912118, 7546.16542346, 7546.16172573, 7546.15802801, 7546.15433028, 7546.15063256, 7546.14693483, 7546.14323711, 7546.13953939, 7546.13584166, 7546.13214394, 7546.12844622, 7546.12474851, 7546.12105078, 7546.11735305, 7546.11365533, 7546.10995761, 7546.10625988, 7546.10256216, 7546.09886444, 7546.09516671, 7546.09146898, 7546.08777126, 7546.08407354, 7546.08037582, 7546.07667809, 7546.07298037, 7546.06928265, 7546.06558 , 7546.06184285, 7546.0581057 , 7546.05436856, 7546.05063141, 7546.04689426, 7546.04315712, 7546.03941997, 7546.03568283, 7546.03194568, 7546.02820853, 7546.02447139, 7546.02073424, 7546.0169971 , 7546.01325995, 7546.0095228 , 7546.00578566, 7546.00204851, 7545.99831137, 7545.99457422, 7545.99083707, 7545.98709993, 7545.98336278, 7545.97962563, 7545.9758885 , 7545.97215134, 7545.96841419, 7545.96467705, 7545.9609399 , ... 7544.57729316, 7544.57328691, 7544.56928068, 7544.56527444, 7544.56126821, 7544.55726197, 7544.55325574, 7544.54924951, 7544.54524327, 7544.54123703, 7544.53723079, 7544.53322455, 7544.52921832, 7544.52521208, 7544.52120584, 7544.51719962, 7544.51319338, 7544.50918714, 7544.50518091, 7544.50117467, 7544.49716844, 7544.4931622 , 7544.48915597, 7544.48514973, 7544.48114349, 7544.47713726, 7544.47312885, 7544.46909368, 7544.4650585 , 7544.46102333, 7544.45698815, 7544.45295298, 7544.44891781, 7544.44488264, 7544.44084747, 7544.4368123 , 7544.43277711, 7544.42874194, 7544.42470675, 7544.4206716 , 7544.41663642, 7544.41260124, 7544.40856608, 7544.40453091, 7544.40049571, 7544.39646055, 7544.39242537, 7544.3883902 , 7544.38435503, 7544.38031985, 7544.37628468, 7544.37224951, 7544.36821434, 7544.36417916, 7544.36014399, 7544.35610881, 7544.35207364, 7544.34803848, 7544.34400329, 7544.33996812, 7544.33593295, 7544.33189777, 7544.32786259, 7544.32382742, 7544.31979225, 7544.31575708, 7544.31172191, 7544.30768673, 7544.30365155, 7544.29961638, 7544.29558121, 7544.29154603, 7544.28751086, 7544.28347569, 7544.27944051, 7544.27540534, 7544.27137017])
- ground_heading(line, sample)float32dask.array<chunksize=(513, 530), meta=np.ndarray>
- comment :
- at ground level, computed from lon/lat in azimuth direction
- long_name :
- Platform heading (azimuth from North)
- units :
- Degrees
Array Chunk Bytes 1.04 MiB 1.04 MiB Shape (513, 530) (513, 530) Dask graph 1 chunks in 4 graph layers Data type float32 numpy.ndarray - time(line)datetime64[ns]dask.array<chunksize=(513,), meta=np.ndarray>
Array Chunk Bytes 4.01 kiB 4.01 kiB Shape (513,) (513,) Dask graph 1 chunks in 5 graph layers Data type datetime64[ns] numpy.ndarray - latitude(line, sample)float64dask.array<chunksize=(513, 530), meta=np.ndarray>
- history :
- /product/imageAttributes/geographicInformation/geolocationGrid/imageTiePoint/geodeticCoordinate/latitude
Array Chunk Bytes 2.07 MiB 2.07 MiB Shape (513, 530) (513, 530) Dask graph 1 chunks in 4 graph layers Data type float64 numpy.ndarray - longitude(line, sample)float64dask.array<chunksize=(513, 530), meta=np.ndarray>
- history :
- /product/imageAttributes/geographicInformation/geolocationGrid/imageTiePoint/geodeticCoordinate/longitude
Array Chunk Bytes 2.07 MiB 2.07 MiB Shape (513, 530) (513, 530) Dask graph 1 chunks in 4 graph layers Data type float64 numpy.ndarray - altitude(line, sample)float64dask.array<chunksize=(513, 530), meta=np.ndarray>
- history :
- /product/imageAttributes/geographicInformation/geolocationGrid/imageTiePoint/geodeticCoordinate/height
Array Chunk Bytes 2.07 MiB 2.07 MiB Shape (513, 530) (513, 530) Dask graph 1 chunks in 4 graph layers Data type float64 numpy.ndarray - incidence(line, sample)float64dask.array<chunksize=(513, 530), meta=np.ndarray>
Array Chunk Bytes 2.07 MiB 2.07 MiB Shape (513, 530) (513, 530) Dask graph 1 chunks in 37 graph layers Data type float64 numpy.ndarray - elevation(line, sample)float64dask.array<chunksize=(513, 530), meta=np.ndarray>
Array Chunk Bytes 2.07 MiB 2.07 MiB Shape (513, 530) (513, 530) Dask graph 1 chunks in 43 graph layers Data type float64 numpy.ndarray
- linePandasIndex
PandasIndex(Index([ 9.5, 29.5, 49.5, 69.5, 89.5, 109.5, 129.5, 149.5, 169.5, 189.5, ... 10069.5, 10089.5, 10109.5, 10129.5, 10149.5, 10169.5, 10189.5, 10209.5, 10229.5, 10249.5], dtype='float64', name='line', length=513))
- polPandasIndex
PandasIndex(Index(['VV', 'VH'], dtype='object', name='pol'))
- samplePandasIndex
PandasIndex(Index([ 9.5, 29.5, 49.5, 69.5, 89.5, 109.5, 129.5, 149.5, 169.5, 189.5, ... 10409.5, 10429.5, 10449.5, 10469.5, 10489.5, 10509.5, 10529.5, 10549.5, 10569.5, 10589.5], dtype='float64', name='sample', length=530))
- product_path :
- /home1/scratch/agrouaze/xsardatasync/xsardata/RS2_OK135107_PK1187782_DK1151894_SCWA_20220407_182127_VV_VH_SGF
- satellite :
- RADARSAT-2
- inputDatasetId :
- /Fred/RSAT-2/610044P
- rawDataStartTime :
- 2022-04-07T18:21:27.688416000
- satelliteHeight_units :
- m
- satelliteHeight :
- 800612.0083192665
- passDirection :
- Descending
- swath :
- SCWA
- product :
- SGF
- pols :
- VH VV
- name :
- RADARSAT2_DS:/home1/scratch/agrouaze/xsardatasync/xsardata/RS2_OK135107_PK1187782_DK1151894_SCWA_20220407_182127_VV_VH_SGF:
- start_date :
- 2022-04-07 18:21:27.401672
- stop_date :
- 2022-04-07 18:22:44.030964
- footprint :
- POLYGON ((168.8147284622835 -19.82390747944243, 163.9252572974075 -18.62823753782336, 162.6387987182235 -23.10230390032916, 167.6676039112585 -24.34397982283393, 168.8147284622835 -19.82390747944243))
- coverage :
- 515km * 530km (line * sample )
- pixel_line_m :
- 50.0
- pixel_sample_m :
- 50.0
- approx_transform :
- |-0.00,-0.00, 168.85| |-0.00, 0.00,-19.85| | 0.00, 0.00, 1.00|
Variables lines_flipped
and samples_flipped
are added to the dataset to know if these have been flipped (in order to follow xsar convention)
Alternatives solutions to open dataset and datatree
[10]:
# Open dataset
xsar.open_dataset(path, resolution=resolution)
/home/datawork-cersat-public/cache/project/mpc-sentinel1/workspace/mamba/envs/testgeoviewsnumpy/lib/python3.11/site-packages/xradarsat2/radarSat2_xarray_reader.py:299: UserWarning: no explicit representation of timezones available for np.datetime64
timestamp.append(np.datetime64(value["timeStamp"]).astype("datetime64[ns]"))
/home/datawork-cersat-public/cache/project/mpc-sentinel1/workspace/mamba/envs/testgeoviewsnumpy/lib/python3.11/site-packages/xradarsat2/radarSat2_xarray_reader.py:462: UserWarning: no explicit representation of timezones available for np.datetime64
timestamp.append(np.datetime64(value["timeStamp"]).astype("datetime64[ns]"))
/home/datawork-cersat-public/cache/project/mpc-sentinel1/workspace/mamba/envs/testgeoviewsnumpy/lib/python3.11/site-packages/xradarsat2/radarSat2_xarray_reader.py:570: UserWarning: no explicit representation of timezones available for np.datetime64
times.append(np.datetime64(value["timeOfDopplerCentroidEstimate"]).astype("datetime64[ns]"))
/home/datawork-cersat-public/cache/project/mpc-sentinel1/workspace/mamba/envs/testgeoviewsnumpy/lib/python3.11/site-packages/xradarsat2/radarSat2_xarray_reader.py:2056: UserWarning: no explicit representation of timezones available for np.datetime64
final_dic[key] = np.datetime64(dic[key]).astype("datetime64[ns]")
[10]:
<xarray.Dataset> Size: 46MB Dimensions: (line: 513, pol: 2, sample: 530) Coordinates: * line (line) float64 4kB 9.5 29.5 49.5 ... 1.023e+04 1.025e+04 * pol (pol) <U2 16B 'VV' 'VH' * sample (sample) float64 4kB 9.5 29.5 49.5 ... 1.057e+04 1.059e+04 Data variables: (12/23) digital_number (pol, line, sample) uint16 1MB dask.array<chunksize=(1, 513, 530), meta=np.ndarray> lines_flipped bool 1B False samples_flipped bool 1B True sampleSpacing float64 8B 1e+03 lineSpacing float64 8B 1e+03 sigma0_raw (pol, line, sample) float64 4MB dask.array<chunksize=(1, 513, 530), meta=np.ndarray> ... ... time (line) datetime64[ns] 4kB dask.array<chunksize=(513,), meta=np.ndarray> latitude (line, sample) float64 2MB dask.array<chunksize=(513, 530), meta=np.ndarray> longitude (line, sample) float64 2MB dask.array<chunksize=(513, 530), meta=np.ndarray> altitude (line, sample) float64 2MB dask.array<chunksize=(513, 530), meta=np.ndarray> incidence (line, sample) float64 2MB dask.array<chunksize=(513, 530), meta=np.ndarray> elevation (line, sample) float64 2MB dask.array<chunksize=(513, 530), meta=np.ndarray> Attributes: (12/18) product_path: /home1/scratch/agrouaze/xsardatasync/xsardata/RS2... satellite: RADARSAT-2 inputDatasetId: /Fred/RSAT-2/610044P rawDataStartTime: 2022-04-07T18:21:27.688416000 satelliteHeight_units: m satelliteHeight: 800612.0083192665 ... ... stop_date: 2022-04-07 18:22:44.030964 footprint: POLYGON ((168.8147284622835 -19.82390747944243, 1... coverage: 515km * 530km (line * sample ) pixel_line_m: 50.0 pixel_sample_m: 50.0 approx_transform: |-0.00,-0.00, 168.85|\n|-0.00, 0.00,-19.85|\n| 0....
- line: 513
- pol: 2
- sample: 530
- line(line)float649.5 29.5 ... 1.023e+04 1.025e+04
array([9.50000e+00, 2.95000e+01, 4.95000e+01, ..., 1.02095e+04, 1.02295e+04, 1.02495e+04])
- pol(pol)<U2'VV' 'VH'
array(['VV', 'VH'], dtype='<U2')
- sample(sample)float649.5 29.5 ... 1.057e+04 1.059e+04
array([9.50000e+00, 2.95000e+01, 4.95000e+01, ..., 1.05495e+04, 1.05695e+04, 1.05895e+04])
- digital_number(pol, line, sample)uint16dask.array<chunksize=(1, 513, 530), meta=np.ndarray>
- comment :
- not denoised digital number, resampled at "1000m" with rasterio.enums.Resampling.rms
- history :
- digital_number: imagery_V*.tif
Array Chunk Bytes 1.04 MiB 531.04 kiB Shape (2, 513, 530) (1, 513, 530) Dask graph 2 chunks in 4 graph layers Data type uint16 numpy.ndarray - lines_flipped()boolFalse
- meaning :
- xsar convention : increasing time along line axis (whatever ascending or descending pass direction)
array(False)
- samples_flipped()boolTrue
- meaning :
- xsar convention : increasing incidence values along samples axis
array(True)
- sampleSpacing()float641e+03
- units :
- m
- referential :
- ground
array(1000.)
- lineSpacing()float641e+03
- units :
- m
array(1000.)
- sigma0_raw(pol, line, sample)float64dask.array<chunksize=(1, 513, 530), meta=np.ndarray>
Array Chunk Bytes 4.15 MiB 2.07 MiB Shape (2, 513, 530) (1, 513, 530) Dask graph 2 chunks in 20 graph layers Data type float64 numpy.ndarray - nesz(pol, line, sample)float32dask.array<chunksize=(1, 513, 530), meta=np.ndarray>
Array Chunk Bytes 2.07 MiB 1.04 MiB Shape (2, 513, 530) (1, 513, 530) Dask graph 2 chunks in 11 graph layers Data type float32 numpy.ndarray - gamma0_raw(pol, line, sample)float64dask.array<chunksize=(1, 513, 530), meta=np.ndarray>
Array Chunk Bytes 4.15 MiB 2.07 MiB Shape (2, 513, 530) (1, 513, 530) Dask graph 2 chunks in 20 graph layers Data type float64 numpy.ndarray - negz(pol, line, sample)float32dask.array<chunksize=(1, 513, 530), meta=np.ndarray>
Array Chunk Bytes 2.07 MiB 1.04 MiB Shape (2, 513, 530) (1, 513, 530) Dask graph 2 chunks in 11 graph layers Data type float32 numpy.ndarray - beta0_raw(pol, line, sample)float64dask.array<chunksize=(1, 513, 530), meta=np.ndarray>
Array Chunk Bytes 4.15 MiB 2.07 MiB Shape (2, 513, 530) (1, 513, 530) Dask graph 2 chunks in 20 graph layers Data type float64 numpy.ndarray - nebz(pol, line, sample)float32dask.array<chunksize=(1, 513, 530), meta=np.ndarray>
Array Chunk Bytes 2.07 MiB 1.04 MiB Shape (2, 513, 530) (1, 513, 530) Dask graph 2 chunks in 11 graph layers Data type float32 numpy.ndarray - sigma0(pol, line, sample)float64dask.array<chunksize=(1, 513, 530), meta=np.ndarray>
- comment :
- not clipped, some values can be <0
Array Chunk Bytes 4.15 MiB 2.07 MiB Shape (2, 513, 530) (1, 513, 530) Dask graph 2 chunks in 31 graph layers Data type float64 numpy.ndarray - beta0(pol, line, sample)float64dask.array<chunksize=(1, 513, 530), meta=np.ndarray>
- comment :
- not clipped, some values can be <0
Array Chunk Bytes 4.15 MiB 2.07 MiB Shape (2, 513, 530) (1, 513, 530) Dask graph 2 chunks in 31 graph layers Data type float64 numpy.ndarray - gamma0(pol, line, sample)float64dask.array<chunksize=(1, 513, 530), meta=np.ndarray>
- comment :
- not clipped, some values can be <0
Array Chunk Bytes 4.15 MiB 2.07 MiB Shape (2, 513, 530) (1, 513, 530) Dask graph 2 chunks in 31 graph layers Data type float64 numpy.ndarray - land_mask(line, sample)int8dask.array<chunksize=(513, 530), meta=np.ndarray>
- history :
- land_mask: cartopy.feature.NaturalEarthFeature land
- meaning :
- 0: ocean , 1: land
Array Chunk Bytes 265.52 kiB 265.52 kiB Shape (513, 530) (513, 530) Dask graph 1 chunks in 3 graph layers Data type int8 numpy.ndarray - velocity(line)float647.546e+03 7.546e+03 ... 7.544e+03
array([7546.25416882, 7546.25047109, 7546.24677337, 7546.24307564, 7546.23937792, 7546.2356802 , 7546.23198248, 7546.22828475, 7546.22458703, 7546.22088931, 7546.21719158, 7546.21349386, 7546.20979613, 7546.20609842, 7546.20240069, 7546.19870297, 7546.19500524, 7546.19130752, 7546.18760979, 7546.18391207, 7546.18021435, 7546.17651663, 7546.1728189 , 7546.16912118, 7546.16542346, 7546.16172573, 7546.15802801, 7546.15433028, 7546.15063256, 7546.14693483, 7546.14323711, 7546.13953939, 7546.13584166, 7546.13214394, 7546.12844622, 7546.12474851, 7546.12105078, 7546.11735305, 7546.11365533, 7546.10995761, 7546.10625988, 7546.10256216, 7546.09886444, 7546.09516671, 7546.09146898, 7546.08777126, 7546.08407354, 7546.08037582, 7546.07667809, 7546.07298037, 7546.06928265, 7546.06558 , 7546.06184285, 7546.0581057 , 7546.05436856, 7546.05063141, 7546.04689426, 7546.04315712, 7546.03941997, 7546.03568283, 7546.03194568, 7546.02820853, 7546.02447139, 7546.02073424, 7546.0169971 , 7546.01325995, 7546.0095228 , 7546.00578566, 7546.00204851, 7545.99831137, 7545.99457422, 7545.99083707, 7545.98709993, 7545.98336278, 7545.97962563, 7545.9758885 , 7545.97215134, 7545.96841419, 7545.96467705, 7545.9609399 , ... 7544.57729316, 7544.57328691, 7544.56928068, 7544.56527444, 7544.56126821, 7544.55726197, 7544.55325574, 7544.54924951, 7544.54524327, 7544.54123703, 7544.53723079, 7544.53322455, 7544.52921832, 7544.52521208, 7544.52120584, 7544.51719962, 7544.51319338, 7544.50918714, 7544.50518091, 7544.50117467, 7544.49716844, 7544.4931622 , 7544.48915597, 7544.48514973, 7544.48114349, 7544.47713726, 7544.47312885, 7544.46909368, 7544.4650585 , 7544.46102333, 7544.45698815, 7544.45295298, 7544.44891781, 7544.44488264, 7544.44084747, 7544.4368123 , 7544.43277711, 7544.42874194, 7544.42470675, 7544.4206716 , 7544.41663642, 7544.41260124, 7544.40856608, 7544.40453091, 7544.40049571, 7544.39646055, 7544.39242537, 7544.3883902 , 7544.38435503, 7544.38031985, 7544.37628468, 7544.37224951, 7544.36821434, 7544.36417916, 7544.36014399, 7544.35610881, 7544.35207364, 7544.34803848, 7544.34400329, 7544.33996812, 7544.33593295, 7544.33189777, 7544.32786259, 7544.32382742, 7544.31979225, 7544.31575708, 7544.31172191, 7544.30768673, 7544.30365155, 7544.29961638, 7544.29558121, 7544.29154603, 7544.28751086, 7544.28347569, 7544.27944051, 7544.27540534, 7544.27137017])
- ground_heading(line, sample)float32dask.array<chunksize=(513, 530), meta=np.ndarray>
- comment :
- at ground level, computed from lon/lat in azimuth direction
- long_name :
- Platform heading (azimuth from North)
- units :
- Degrees
Array Chunk Bytes 1.04 MiB 1.04 MiB Shape (513, 530) (513, 530) Dask graph 1 chunks in 4 graph layers Data type float32 numpy.ndarray - time(line)datetime64[ns]dask.array<chunksize=(513,), meta=np.ndarray>
Array Chunk Bytes 4.01 kiB 4.01 kiB Shape (513,) (513,) Dask graph 1 chunks in 5 graph layers Data type datetime64[ns] numpy.ndarray - latitude(line, sample)float64dask.array<chunksize=(513, 530), meta=np.ndarray>
- history :
- /product/imageAttributes/geographicInformation/geolocationGrid/imageTiePoint/geodeticCoordinate/latitude
Array Chunk Bytes 2.07 MiB 2.07 MiB Shape (513, 530) (513, 530) Dask graph 1 chunks in 4 graph layers Data type float64 numpy.ndarray - longitude(line, sample)float64dask.array<chunksize=(513, 530), meta=np.ndarray>
- history :
- /product/imageAttributes/geographicInformation/geolocationGrid/imageTiePoint/geodeticCoordinate/longitude
Array Chunk Bytes 2.07 MiB 2.07 MiB Shape (513, 530) (513, 530) Dask graph 1 chunks in 4 graph layers Data type float64 numpy.ndarray - altitude(line, sample)float64dask.array<chunksize=(513, 530), meta=np.ndarray>
- history :
- /product/imageAttributes/geographicInformation/geolocationGrid/imageTiePoint/geodeticCoordinate/height
Array Chunk Bytes 2.07 MiB 2.07 MiB Shape (513, 530) (513, 530) Dask graph 1 chunks in 4 graph layers Data type float64 numpy.ndarray - incidence(line, sample)float64dask.array<chunksize=(513, 530), meta=np.ndarray>
Array Chunk Bytes 2.07 MiB 2.07 MiB Shape (513, 530) (513, 530) Dask graph 1 chunks in 37 graph layers Data type float64 numpy.ndarray - elevation(line, sample)float64dask.array<chunksize=(513, 530), meta=np.ndarray>
Array Chunk Bytes 2.07 MiB 2.07 MiB Shape (513, 530) (513, 530) Dask graph 1 chunks in 43 graph layers Data type float64 numpy.ndarray
- linePandasIndex
PandasIndex(Index([ 9.5, 29.5, 49.5, 69.5, 89.5, 109.5, 129.5, 149.5, 169.5, 189.5, ... 10069.5, 10089.5, 10109.5, 10129.5, 10149.5, 10169.5, 10189.5, 10209.5, 10229.5, 10249.5], dtype='float64', name='line', length=513))
- polPandasIndex
PandasIndex(Index(['VV', 'VH'], dtype='object', name='pol'))
- samplePandasIndex
PandasIndex(Index([ 9.5, 29.5, 49.5, 69.5, 89.5, 109.5, 129.5, 149.5, 169.5, 189.5, ... 10409.5, 10429.5, 10449.5, 10469.5, 10489.5, 10509.5, 10529.5, 10549.5, 10569.5, 10589.5], dtype='float64', name='sample', length=530))
- product_path :
- /home1/scratch/agrouaze/xsardatasync/xsardata/RS2_OK135107_PK1187782_DK1151894_SCWA_20220407_182127_VV_VH_SGF
- satellite :
- RADARSAT-2
- inputDatasetId :
- /Fred/RSAT-2/610044P
- rawDataStartTime :
- 2022-04-07T18:21:27.688416000
- satelliteHeight_units :
- m
- satelliteHeight :
- 800612.0083192665
- passDirection :
- Descending
- swath :
- SCWA
- product :
- SGF
- pols :
- VH VV
- name :
- RADARSAT2_DS:/home1/scratch/agrouaze/xsardatasync/xsardata/RS2_OK135107_PK1187782_DK1151894_SCWA_20220407_182127_VV_VH_SGF:
- start_date :
- 2022-04-07 18:21:27.401672
- stop_date :
- 2022-04-07 18:22:44.030964
- footprint :
- POLYGON ((168.8147284622835 -19.82390747944243, 163.9252572974075 -18.62823753782336, 162.6387987182235 -23.10230390032916, 167.6676039112585 -24.34397982283393, 168.8147284622835 -19.82390747944243))
- coverage :
- 515km * 530km (line * sample )
- pixel_line_m :
- 50.0
- pixel_sample_m :
- 50.0
- approx_transform :
- |-0.00,-0.00, 168.85| |-0.00, 0.00,-19.85| | 0.00, 0.00, 1.00|
[11]:
# Open datatree
xsar.open_datatree(path, resolution=resolution)
/home/datawork-cersat-public/cache/project/mpc-sentinel1/workspace/mamba/envs/testgeoviewsnumpy/lib/python3.11/site-packages/xradarsat2/radarSat2_xarray_reader.py:299: UserWarning: no explicit representation of timezones available for np.datetime64
timestamp.append(np.datetime64(value["timeStamp"]).astype("datetime64[ns]"))
/home/datawork-cersat-public/cache/project/mpc-sentinel1/workspace/mamba/envs/testgeoviewsnumpy/lib/python3.11/site-packages/xradarsat2/radarSat2_xarray_reader.py:462: UserWarning: no explicit representation of timezones available for np.datetime64
timestamp.append(np.datetime64(value["timeStamp"]).astype("datetime64[ns]"))
/home/datawork-cersat-public/cache/project/mpc-sentinel1/workspace/mamba/envs/testgeoviewsnumpy/lib/python3.11/site-packages/xradarsat2/radarSat2_xarray_reader.py:570: UserWarning: no explicit representation of timezones available for np.datetime64
times.append(np.datetime64(value["timeOfDopplerCentroidEstimate"]).astype("datetime64[ns]"))
/home/datawork-cersat-public/cache/project/mpc-sentinel1/workspace/mamba/envs/testgeoviewsnumpy/lib/python3.11/site-packages/xradarsat2/radarSat2_xarray_reader.py:2056: UserWarning: no explicit representation of timezones available for np.datetime64
final_dic[key] = np.datetime64(dic[key]).astype("datetime64[ns]")
[11]:
<xarray.DatasetView> Size: 0B Dimensions: () Data variables: *empty* Attributes: (12/18) product_path: /home1/scratch/agrouaze/xsardatasync/xsardata/RS2... satellite: RADARSAT-2 inputDatasetId: /Fred/RSAT-2/610044P rawDataStartTime: 2022-04-07T18:21:27.688416000 satelliteHeight_units: m satelliteHeight: 800612.0083192665 ... ... stop_date: 2022-04-07 18:22:44.030964 footprint: POLYGON ((168.8147284622835 -19.82390747944243, 1... coverage: 515km * 530km (line * sample ) pixel_line_m: 50.0 pixel_sample_m: 50.0 approx_transform: |-0.00,-0.00, 168.85|\n|-0.00, 0.00,-19.85|\n| 0....
- line: 513
- pol: 2
- sample: 530
- line(line)float649.5 29.5 ... 1.023e+04 1.025e+04
array([9.50000e+00, 2.95000e+01, 4.95000e+01, ..., 1.02095e+04, 1.02295e+04, 1.02495e+04])
- pol(pol)<U2'VV' 'VH'
array(['VV', 'VH'], dtype='<U2')
- sample(sample)float649.5 29.5 ... 1.057e+04 1.059e+04
array([9.50000e+00, 2.95000e+01, 4.95000e+01, ..., 1.05495e+04, 1.05695e+04, 1.05895e+04])
- digital_number(pol, line, sample)uint16dask.array<chunksize=(1, 513, 530), meta=np.ndarray>
- comment :
- not denoised digital number, resampled at "1000m" with rasterio.enums.Resampling.rms
- history :
- digital_number: imagery_V*.tif
Array Chunk Bytes 1.04 MiB 531.04 kiB Shape (2, 513, 530) (1, 513, 530) Dask graph 2 chunks in 4 graph layers Data type uint16 numpy.ndarray - lines_flipped()boolFalse
- meaning :
- xsar convention : increasing time along line axis (whatever ascending or descending pass direction)
array(False)
- samples_flipped()boolTrue
- meaning :
- xsar convention : increasing incidence values along samples axis
array(True)
- sampleSpacing()float641e+03
- units :
- m
- referential :
- ground
array(1000.)
- lineSpacing()float641e+03
- units :
- m
array(1000.)
- sigma0_raw(pol, line, sample)float64dask.array<chunksize=(1, 513, 530), meta=np.ndarray>
Array Chunk Bytes 4.15 MiB 2.07 MiB Shape (2, 513, 530) (1, 513, 530) Dask graph 2 chunks in 20 graph layers Data type float64 numpy.ndarray - nesz(pol, line, sample)float32dask.array<chunksize=(1, 513, 530), meta=np.ndarray>
Array Chunk Bytes 2.07 MiB 1.04 MiB Shape (2, 513, 530) (1, 513, 530) Dask graph 2 chunks in 11 graph layers Data type float32 numpy.ndarray - gamma0_raw(pol, line, sample)float64dask.array<chunksize=(1, 513, 530), meta=np.ndarray>
Array Chunk Bytes 4.15 MiB 2.07 MiB Shape (2, 513, 530) (1, 513, 530) Dask graph 2 chunks in 20 graph layers Data type float64 numpy.ndarray - negz(pol, line, sample)float32dask.array<chunksize=(1, 513, 530), meta=np.ndarray>
Array Chunk Bytes 2.07 MiB 1.04 MiB Shape (2, 513, 530) (1, 513, 530) Dask graph 2 chunks in 11 graph layers Data type float32 numpy.ndarray - beta0_raw(pol, line, sample)float64dask.array<chunksize=(1, 513, 530), meta=np.ndarray>
Array Chunk Bytes 4.15 MiB 2.07 MiB Shape (2, 513, 530) (1, 513, 530) Dask graph 2 chunks in 20 graph layers Data type float64 numpy.ndarray - nebz(pol, line, sample)float32dask.array<chunksize=(1, 513, 530), meta=np.ndarray>
Array Chunk Bytes 2.07 MiB 1.04 MiB Shape (2, 513, 530) (1, 513, 530) Dask graph 2 chunks in 11 graph layers Data type float32 numpy.ndarray - sigma0(pol, line, sample)float64dask.array<chunksize=(1, 513, 530), meta=np.ndarray>
- comment :
- not clipped, some values can be <0
Array Chunk Bytes 4.15 MiB 2.07 MiB Shape (2, 513, 530) (1, 513, 530) Dask graph 2 chunks in 31 graph layers Data type float64 numpy.ndarray - beta0(pol, line, sample)float64dask.array<chunksize=(1, 513, 530), meta=np.ndarray>
- comment :
- not clipped, some values can be <0
Array Chunk Bytes 4.15 MiB 2.07 MiB Shape (2, 513, 530) (1, 513, 530) Dask graph 2 chunks in 31 graph layers Data type float64 numpy.ndarray - gamma0(pol, line, sample)float64dask.array<chunksize=(1, 513, 530), meta=np.ndarray>
- comment :
- not clipped, some values can be <0
Array Chunk Bytes 4.15 MiB 2.07 MiB Shape (2, 513, 530) (1, 513, 530) Dask graph 2 chunks in 31 graph layers Data type float64 numpy.ndarray - land_mask(line, sample)int8dask.array<chunksize=(513, 530), meta=np.ndarray>
- history :
- land_mask: cartopy.feature.NaturalEarthFeature land
- meaning :
- 0: ocean , 1: land
Array Chunk Bytes 265.52 kiB 265.52 kiB Shape (513, 530) (513, 530) Dask graph 1 chunks in 3 graph layers Data type int8 numpy.ndarray - velocity(line)float647.546e+03 7.546e+03 ... 7.544e+03
array([7546.25416882, 7546.25047109, 7546.24677337, 7546.24307564, 7546.23937792, 7546.2356802 , 7546.23198248, 7546.22828475, 7546.22458703, 7546.22088931, 7546.21719158, 7546.21349386, 7546.20979613, 7546.20609842, 7546.20240069, 7546.19870297, 7546.19500524, 7546.19130752, 7546.18760979, 7546.18391207, 7546.18021435, 7546.17651663, 7546.1728189 , 7546.16912118, 7546.16542346, 7546.16172573, 7546.15802801, 7546.15433028, 7546.15063256, 7546.14693483, 7546.14323711, 7546.13953939, 7546.13584166, 7546.13214394, 7546.12844622, 7546.12474851, 7546.12105078, 7546.11735305, 7546.11365533, 7546.10995761, 7546.10625988, 7546.10256216, 7546.09886444, 7546.09516671, 7546.09146898, 7546.08777126, 7546.08407354, 7546.08037582, 7546.07667809, 7546.07298037, 7546.06928265, 7546.06558 , 7546.06184285, 7546.0581057 , 7546.05436856, 7546.05063141, 7546.04689426, 7546.04315712, 7546.03941997, 7546.03568283, 7546.03194568, 7546.02820853, 7546.02447139, 7546.02073424, 7546.0169971 , 7546.01325995, 7546.0095228 , 7546.00578566, 7546.00204851, 7545.99831137, 7545.99457422, 7545.99083707, 7545.98709993, 7545.98336278, 7545.97962563, 7545.9758885 , 7545.97215134, 7545.96841419, 7545.96467705, 7545.9609399 , ... 7544.57729316, 7544.57328691, 7544.56928068, 7544.56527444, 7544.56126821, 7544.55726197, 7544.55325574, 7544.54924951, 7544.54524327, 7544.54123703, 7544.53723079, 7544.53322455, 7544.52921832, 7544.52521208, 7544.52120584, 7544.51719962, 7544.51319338, 7544.50918714, 7544.50518091, 7544.50117467, 7544.49716844, 7544.4931622 , 7544.48915597, 7544.48514973, 7544.48114349, 7544.47713726, 7544.47312885, 7544.46909368, 7544.4650585 , 7544.46102333, 7544.45698815, 7544.45295298, 7544.44891781, 7544.44488264, 7544.44084747, 7544.4368123 , 7544.43277711, 7544.42874194, 7544.42470675, 7544.4206716 , 7544.41663642, 7544.41260124, 7544.40856608, 7544.40453091, 7544.40049571, 7544.39646055, 7544.39242537, 7544.3883902 , 7544.38435503, 7544.38031985, 7544.37628468, 7544.37224951, 7544.36821434, 7544.36417916, 7544.36014399, 7544.35610881, 7544.35207364, 7544.34803848, 7544.34400329, 7544.33996812, 7544.33593295, 7544.33189777, 7544.32786259, 7544.32382742, 7544.31979225, 7544.31575708, 7544.31172191, 7544.30768673, 7544.30365155, 7544.29961638, 7544.29558121, 7544.29154603, 7544.28751086, 7544.28347569, 7544.27944051, 7544.27540534, 7544.27137017])
- ground_heading(line, sample)float32dask.array<chunksize=(513, 530), meta=np.ndarray>
- comment :
- at ground level, computed from lon/lat in azimuth direction
- long_name :
- Platform heading (azimuth from North)
- units :
- Degrees
Array Chunk Bytes 1.04 MiB 1.04 MiB Shape (513, 530) (513, 530) Dask graph 1 chunks in 4 graph layers Data type float32 numpy.ndarray - time(line)datetime64[ns]dask.array<chunksize=(513,), meta=np.ndarray>
Array Chunk Bytes 4.01 kiB 4.01 kiB Shape (513,) (513,) Dask graph 1 chunks in 5 graph layers Data type datetime64[ns] numpy.ndarray - latitude(line, sample)float64dask.array<chunksize=(513, 530), meta=np.ndarray>
- history :
- /product/imageAttributes/geographicInformation/geolocationGrid/imageTiePoint/geodeticCoordinate/latitude
Array Chunk Bytes 2.07 MiB 2.07 MiB Shape (513, 530) (513, 530) Dask graph 1 chunks in 4 graph layers Data type float64 numpy.ndarray - longitude(line, sample)float64dask.array<chunksize=(513, 530), meta=np.ndarray>
- history :
- /product/imageAttributes/geographicInformation/geolocationGrid/imageTiePoint/geodeticCoordinate/longitude
Array Chunk Bytes 2.07 MiB 2.07 MiB Shape (513, 530) (513, 530) Dask graph 1 chunks in 4 graph layers Data type float64 numpy.ndarray - altitude(line, sample)float64dask.array<chunksize=(513, 530), meta=np.ndarray>
- history :
- /product/imageAttributes/geographicInformation/geolocationGrid/imageTiePoint/geodeticCoordinate/height
Array Chunk Bytes 2.07 MiB 2.07 MiB Shape (513, 530) (513, 530) Dask graph 1 chunks in 4 graph layers Data type float64 numpy.ndarray - incidence(line, sample)float64dask.array<chunksize=(513, 530), meta=np.ndarray>
Array Chunk Bytes 2.07 MiB 2.07 MiB Shape (513, 530) (513, 530) Dask graph 1 chunks in 37 graph layers Data type float64 numpy.ndarray - elevation(line, sample)float64dask.array<chunksize=(513, 530), meta=np.ndarray>
Array Chunk Bytes 2.07 MiB 2.07 MiB Shape (513, 530) (513, 530) Dask graph 1 chunks in 43 graph layers Data type float64 numpy.ndarray
<xarray.DatasetView> Size: 46MB Dimensions: (line: 513, pol: 2, sample: 530) Coordinates: * line (line) float64 4kB 9.5 29.5 49.5 ... 1.023e+04 1.025e+04 * pol (pol) <U2 16B 'VV' 'VH' * sample (sample) float64 4kB 9.5 29.5 49.5 ... 1.057e+04 1.059e+04 Data variables: (12/23) digital_number (pol, line, sample) uint16 1MB dask.array<chunksize=(1, 513, 530), meta=np.ndarray> lines_flipped bool 1B False samples_flipped bool 1B True sampleSpacing float64 8B 1e+03 lineSpacing float64 8B 1e+03 sigma0_raw (pol, line, sample) float64 4MB dask.array<chunksize=(1, 513, 530), meta=np.ndarray> ... ... time (line) datetime64[ns] 4kB dask.array<chunksize=(513,), meta=np.ndarray> latitude (line, sample) float64 2MB dask.array<chunksize=(513, 530), meta=np.ndarray> longitude (line, sample) float64 2MB dask.array<chunksize=(513, 530), meta=np.ndarray> altitude (line, sample) float64 2MB dask.array<chunksize=(513, 530), meta=np.ndarray> incidence (line, sample) float64 2MB dask.array<chunksize=(513, 530), meta=np.ndarray> elevation (line, sample) float64 2MB dask.array<chunksize=(513, 530), meta=np.ndarray>
measurement- line: 11
- pixel: 11
- line(line)int640 1027 2055 ... 8220 9248 10276
- rasterAttributes_sampledLineSpacing_units :
- m
- rasterAttributes_sampledLineSpacing_value :
- 50.0
array([ 0, 1027, 2055, 3082, 4110, 5138, 6165, 7193, 8220, 9248, 10276])
- pixel(pixel)int640 1061 2123 ... 8493 9555 10617
- rasterAttributes_sampledPixelSpacing_units :
- m
- rasterAttributes_sampledPixelSpacing_value :
- 50.0
array([ 0, 1061, 2123, 3185, 4246, 5308, 6370, 7431, 8493, 9555, 10617])
- latitude(line, pixel)float64-19.82 -19.71 ... -23.23 -23.1
- units :
- deg
- xpath :
- /product/imageAttributes/geographicInformation/geolocationGrid/imageTiePoint/geodeticCoordinate/latitude
- Description :
- Geodetic latitude (units = deg)
array([[-19.82390748, -19.71020793, -19.59518856, -19.47886136, -19.36122117, -19.24226667, -19.1220062 , -19.00045628, -18.87763654, -18.75356264, -18.62823754], [-20.27630107, -20.16231142, -20.04696894, -19.93028583, -19.81225703, -19.69288137, -19.57216733, -19.45013163, -19.32679418, -19.20217088, -19.0762649 ], [-20.7286158 , -20.61433017, -20.49865901, -20.38161455, -20.26319178, -20.14338957, -20.02221652, -19.89968957, -19.77582886, -19.65065054, -19.52415799], [-21.18084653, -21.0662625 , -20.95025957, -20.83285024, -20.7140296 , -20.59379666, -20.4721602 , -20.34913737, -20.22474858, -20.09901026, -19.97192602], [-21.63299412, -21.51810544, -21.40176486, -21.28398491, -21.16476073, -21.04409139, -20.92198579, -20.79846131, -20.67353862, -20.5472344 , -20.41955247], [-22.08505314, -21.96985713, -21.85317556, -21.7350212 , -21.61538931, -21.4942791 , -21.37169966, -21.24766861, -21.12220688, -20.99533146, -20.86704641], [-22.53702463, -22.42151504, -22.3044865 , -22.18595187, -22.06590644, -21.94434951, -21.82129032, -21.69674671, -21.57073989, -21.44328712, -21.3143927 ], [-22.9889047 , -22.87307839, -22.75569914, -22.63678005, -22.51631656, -22.39430811, -22.27076412, -22.14570268, -22.01914531, -21.89110956, -21.76159999], [-23.44069154, -23.32454219, -23.20680616, -23.08749663, -22.96660909, -22.84414307, -22.72010818, -22.59452272, -22.4674085 , -22.33878338, -22.20865218], [-23.89238427, -23.77590827, -23.65781128, -23.53810674, -23.41679026, -23.29386151, -23.16933029, -23.04321519, -22.91553832, -22.78631785, -22.65555886], [-24.34397982, -24.22717071, -24.10870651, -23.98860074, -23.86684907, -23.74345129, -23.61841737, -23.49176614, -23.36352001, -23.23369749, -23.1023039 ]])
- longitude(line, pixel)float64168.8 168.3 167.8 ... 163.1 162.6
- units :
- deg
- xpath :
- /product/imageAttributes/geographicInformation/geolocationGrid/imageTiePoint/geodeticCoordinate/longitude
- Description :
- Geodetic longitude (units = deg)
array([[168.81472846, 168.32249998, 167.83104776, 167.3403784 , 166.85042686, 166.36114675, 165.8725324 , 165.38461077, 164.89741948, 164.41097839, 163.9252573 ], [168.70275313, 168.20919687, 167.71643791, 167.22448332, 166.7332681 , 166.24274597, 165.75291152, 165.26379215, 164.77542596, 164.28783332, 163.8009844 ], [168.59021038, 168.09528215, 167.60117481, 167.10789509, 166.61537758, 166.12357585, 165.63248458, 165.1421315 , 164.65255515, 164.16377635, 163.67576561], [168.4770878 , 167.98075825, 167.48527126, 166.9906341 , 166.49678145, 166.00366702, 165.51128579, 165.01966592, 164.52884651, 164.03884889, 163.54964396], [168.36337334, 167.86559656, 167.36868687, 166.87265114, 166.37742364, 165.8829579 , 165.38924904, 164.89632555, 164.404227 , 163.91297518, 163.42254136], [168.24903707, 167.7497828 , 167.25141823, 166.75395076, 166.25731472, 165.76146379, 165.26639337, 164.77213245, 164.27872114, 163.78618179, 163.29448607], [168.1340763 , 167.63329861, 167.13343584, 166.63449509, 166.13641032, 165.63913507, 165.14266489, 164.64702914, 164.15226843, 163.65840563, 163.16541277], [168.01846251, 167.51612903, 167.01473415, 166.51428544, 166.01471693, 165.51598225, 165.01807728, 164.52103186, 164.02488719, 163.52966671, 163.03534287], [167.90219443, 167.39825892, 166.89528815, 166.39328939, 165.89219631, 165.39196244, 164.89258381, 164.39409068, 163.89652478, 163.39991005, 162.90421938], [167.78524631, 167.27967433, 166.775092 , 166.27150701, 165.76885299, 165.26708358, 164.76619513, 164.26621839, 163.7671957 , 163.2691516 , 162.77205941], [167.66760391, 167.16034863, 166.65411027, 166.1488962 , 165.64463967, 165.1412942 , 164.63885631, 164.13735718, 163.6368397 , 163.13732896, 162.63879872]])
- height(line, pixel)float6419.01 19.01 19.01 ... 19.01 19.01
- units :
- m
- xpath :
- /product/imageAttributes/geographicInformation/geolocationGrid/imageTiePoint/geodeticCoordinate/height
- Description :
- Geodetic height above reference ellipsoid (units = m)
array([[19.00683975, 19.00683975, 19.00683975, 19.00683975, 19.00683975, 19.00683975, 19.00683975, 19.00683975, 19.00683975, 19.00683975, 19.00683975], [19.00683975, 19.00683975, 19.00683975, 19.00683975, 19.00683975, 19.00683975, 19.00683975, 19.00683975, 19.00683975, 19.00683975, 19.00683975], [19.00683975, 19.00683975, 19.00683975, 19.00683975, 19.00683975, 19.00683975, 19.00683975, 19.00683975, 19.00683975, 19.00683975, 19.00683975], [19.00683975, 19.00683975, 19.00683975, 19.00683975, 19.00683975, 19.00683975, 19.00683975, 19.00683975, 19.00683975, 19.00683975, 19.00683975], [19.00683975, 19.00683975, 19.00683975, 19.00683975, 19.00683975, 19.00683975, 19.00683975, 19.00683975, 19.00683975, 19.00683975, 19.00683975], [19.00683975, 19.00683975, 19.00683975, 19.00683975, 19.00683975, 19.00683975, 19.00683975, 19.00683975, 19.00683975, 19.00683975, 19.00683975], [19.00683975, 19.00683975, 19.00683975, 19.00683975, 19.00683975, 19.00683975, 19.00683975, 19.00683975, 19.00683975, 19.00683975, 19.00683975], [19.00683975, 19.00683975, 19.00683975, 19.00683975, 19.00683975, 19.00683975, 19.00683975, 19.00683975, 19.00683975, 19.00683975, 19.00683975], [19.00683975, 19.00683975, 19.00683975, 19.00683975, 19.00683975, 19.00683975, 19.00683975, 19.00683975, 19.00683975, 19.00683975, 19.00683975], [19.00683975, 19.00683975, 19.00683975, 19.00683975, 19.00683975, 19.00683975, 19.00683975, 19.00683975, 19.00683975, 19.00683975, 19.00683975], [19.00683975, 19.00683975, 19.00683975, 19.00683975, 19.00683975, 19.00683975, 19.00683975, 19.00683975, 19.00683975, 19.00683975, 19.00683975]])
- productFormat :
- GeoTIFF
- outputMediaInterleaving :
- BSQ
- rasterAttributes_dataType :
- Magnitude Detected
- rasterAttributes_bitsPerSample_dataStream :
- Magnitude
- rasterAttributes_bitsPerSample_value :
- 16
- rasterAttributes_numberOfSamplesPerLine :
- 10618
- rasterAttributes_numberOfLines :
- 10277
- rasterAttributes_sampledPixelSpacing_units :
- m
- rasterAttributes_sampledPixelSpacing_value :
- 50.0
- rasterAttributes_sampledLineSpacing_units :
- m
- rasterAttributes_sampledLineSpacing_value :
- 50.0
- rasterAttributes_lineTimeOrdering :
- Increasing
- rasterAttributes_pixelTimeOrdering :
- Decreasing
- footprint :
- POLYGON ((168.8147284622835 -19.82390747944243, 163.9252572974075 -18.62823753782336, 162.6387987182235 -23.10230390032916, 167.6676039112585 -24.34397982283393, 168.8147284622835 -19.82390747944243))
- history :
- annotations
<xarray.DatasetView> Size: 3kB Dimensions: (line: 11, pixel: 11) Coordinates: * line (line) int64 88B 0 1027 2055 3082 4110 ... 7193 8220 9248 10276 * pixel (pixel) int64 88B 0 1061 2123 3185 4246 ... 7431 8493 9555 10617 Data variables: latitude (line, pixel) float64 968B -19.82 -19.71 -19.6 ... -23.23 -23.1 longitude (line, pixel) float64 968B 168.8 168.3 167.8 ... 163.1 162.6 height (line, pixel) float64 968B 19.01 19.01 19.01 ... 19.01 19.01 Attributes: (12/15) productFormat: GeoTIFF outputMediaInterleaving: BSQ rasterAttributes_dataType: Magnitude Detected rasterAttributes_bitsPerSample_dataStream: Magnitude rasterAttributes_bitsPerSample_value: 16 rasterAttributes_numberOfSamplesPerLine: 10618 ... ... rasterAttributes_sampledLineSpacing_units: m rasterAttributes_sampledLineSpacing_value: 50.0 rasterAttributes_lineTimeOrdering: Increasing rasterAttributes_pixelTimeOrdering: Decreasing footprint: POLYGON ((168.8147284622835 ... history: annotations
geolocation_annotation- pixel: 10618
- pixel(pixel)int640 1 2 3 ... 10614 10615 10616 10617
array([ 0, 1, 2, ..., 10615, 10616, 10617])
- sigma0_lut(pixel)float644.071e+07 4.07e+07 ... 1.785e+07
- offset :
- 0.0
array([40709550., 40702230., 40694940., ..., 17846740., 17846190., 17845640.])
- gamma0_lut(pixel)float643.838e+07 3.837e+07 ... 1.157e+07
- offset :
- 0.0
array([38376630., 38368870., 38361140., ..., 11576030., 11575180., 11574340.])
- beta0_lut(pixel)float641.358e+07 1.358e+07 ... 1.358e+07
- offset :
- 0.0
array([13583140., 13583140., 13583140., ..., 13583140., 13583140., 13583140.])
- Description :
- RADARSAT Product LUT. (c) COPYRIGHT MacDonald Dettwiler and Associates Ltd., 2003 All Rights Reserved.Three output scaling Look-up Tables (LUTs) are included with every product. These LUTs allow one to convert the digital numbers foundin the output product to sigma-nought, beta-nought, or gamma-noughtvalues (depending on which LUT is used) by applying a constantoffset and range dependent gain to the SAR imagery.There is one entry in the gains list for each range sample in theimagery. In order to convert the digital number of a given rangesample to a calibrated value, the digital value is first squared,then the offset (B) is added and the result is divided by thegain value (A) corresponding to the range sample.i.e., calibrated value = ( digital value^2 + B ) / A
- history :
- annotations
<xarray.DatasetView> Size: 340kB Dimensions: (pixel: 10618) Coordinates: * pixel (pixel) int64 85kB 0 1 2 3 4 5 ... 10613 10614 10615 10616 10617 Data variables: sigma0_lut (pixel) float64 85kB 4.071e+07 4.07e+07 ... 1.785e+07 1.785e+07 gamma0_lut (pixel) float64 85kB 3.838e+07 3.837e+07 ... 1.158e+07 1.157e+07 beta0_lut (pixel) float64 85kB 1.358e+07 1.358e+07 ... 1.358e+07 1.358e+07 Attributes: Description: RADARSAT Product LUT. (c) COPYRIGHT MacDonald Dettwiler and... history: annotations
lut- beam: 4
- pole: 2
- pixel: 99
- beam(beam)<U3'W1' 'W2' 'W30' 'S7'
array(['W1', 'W2', 'W30', 'S7'], dtype='<U3')
- pole(pole)<U2'VH' 'VV'
array(['VH', 'VV'], dtype='<U2')
- pixel(pixel)int640 1 2 3 4 5 6 ... 93 94 95 96 97 98
array([ 0, 1, 2, 3, 4, 5, 6, 7, 8, 9, 10, 11, 12, 13, 14, 15, 16, 17, 18, 19, 20, 21, 22, 23, 24, 25, 26, 27, 28, 29, 30, 31, 32, 33, 34, 35, 36, 37, 38, 39, 40, 41, 42, 43, 44, 45, 46, 47, 48, 49, 50, 51, 52, 53, 54, 55, 56, 57, 58, 59, 60, 61, 62, 63, 64, 65, 66, 67, 68, 69, 70, 71, 72, 73, 74, 75, 76, 77, 78, 79, 80, 81, 82, 83, 84, 85, 86, 87, 88, 89, 90, 91, 92, 93, 94, 95, 96, 97, 98])
- beta0_noise_lut(pixel)float64-22.05 -23.83 ... -26.65 -25.52
- pixelFirstNoiseValue :
- 33
- stepSize :
- 108
- numberOfNoiseLevelValues :
- 99
- xpath :
- /product/sourceAttributes/radarParameters/referenceNoiseLevel/noiseLevelValues
- noiseLevelValues_units :
- dB
- Description :
- Estimated noise level values as a function of georeferenced image pixel position in range. For SSG and SPG products, these apply to intermediate georeferenced image data prior to geocoding. (units = dB)
array([-22.0480309, -23.8308601, -24.6454506, -24.8271008, -24.5917206, -24.2823792, -24.0880699, -24.16012 , -24.3325596, -24.4630699, -24.4747009, -24.3628807, -24.2323208, -24.1418209, -24.1742001, -24.3378296, -24.5296803, -24.6641808, -24.7199593, -24.7104893, -24.6347198, -24.5283108, -24.4534492, -24.5001793, -24.6506805, -24.8547897, -25.1622391, -25.4442997, -25.6879005, -25.6855793, -25.3954391, -24.6379108, -26.3336792, -26.6781902, -26.5182705, -26.0002708, -25.47616 , -24.9876404, -24.7475109, -24.9693298, -25.5615807, -26.2679996, -26.8668308, -27.1953201, -27.1978703, -26.8729706, -26.3505802, -25.7925892, -25.39958 , -25.4009094, -25.7947006, -26.49226 , -27.2579708, -27.8481808, -28.1557598, -28.0971909, -27.62146 , -26.7268295, -25.5062504, -25.5776691, -25.67976 , -25.8911991, -26.2002907, -26.52981 , -26.8151493, -26.9865208, -27.03685 , -26.9238396, -26.7098198, -26.4480991, -26.1808109, -26.0066204, -25.9750309, -26.0835495, -26.2642899, -26.4492493, -26.5925102, -26.5693398, -26.3428802, -28.0349197, -28.3677692, -28.3973598, -28.2351894, -27.9366703, -27.5906696, -27.2849808, -27.0888195, -27.0662594, -27.2208996, -27.5067596, -27.86273 , -28.2094593, -28.4701309, -28.5607796, -28.4260101, -28.05089 , -27.4847393, -26.6474609, -25.5237503])
- sigma0_noise_lut(pixel)float64-26.78 -28.48 ... -27.84 -26.7
- pixelFirstNoiseValue :
- 33
- stepSize :
- 108
- numberOfNoiseLevelValues :
- 99
- xpath :
- /product/sourceAttributes/radarParameters/referenceNoiseLevel/noiseLevelValues
- noiseLevelValues_units :
- dB
- Description :
- Estimated noise level values as a function of georeferenced image pixel position in range. For SSG and SPG products, these apply to intermediate georeferenced image data prior to geocoding. (units = dB)
array([-26.7759094, -28.4762707, -29.2104492, -29.3136806, -29.0017891, -28.6177807, -28.3505707, -28.3514309, -28.4543304, -28.5168991, -28.4621296, -28.2853909, -28.0913601, -27.9387798, -27.9104195, -28.0146294, -28.1483307, -28.2259007, -28.2259407, -28.1618996, -28.0326595, -27.8738995, -27.7477303, -27.7441807, -27.8454094, -28.0012207, -28.2613201, -28.4969406, -28.6950207, -28.6480408, -28.3140793, -27.5135803, -29.1671791, -29.4703102, -29.2697906, -28.7119293, -28.1486893, -27.6217594, -27.3439102, -27.5286903, -28.0845699, -28.75527 , -29.3190193, -29.6130295, -29.5817204, -29.2235508, -28.6684608, -28.0783501, -27.6537609, -27.6240501, -27.9873409, -28.6548996, -29.39114 , -29.9523506, -30.2314301, -30.1448402, -29.6415405, -28.7198105, -27.4725704, -27.5177708, -27.5940495, -27.7801208, -28.0642395, -28.3691998, -28.6303596, -28.7779503, -28.8048706, -28.6688194, -28.4321308, -28.1480999, -27.8588409, -27.6630306, -27.6101398, -27.69771 , -27.8578091, -28.0224609, -28.14571 , -28.1028309, -27.8569794, -29.5299091, -29.8439407, -29.8549995, -29.6745605, -29.3580608, -28.9943409, -28.6711998, -28.45784 , -28.4183407, -28.5562801, -28.8257008, -29.1654491, -29.4962006, -29.7411308, -29.8162594, -29.6662006, -29.2759991, -28.6949902, -27.84305 , -26.7049007])
- gamma0_noise_lut(pixel)float64-26.51 -28.2 ... -25.98 -24.82
- pixelFirstNoiseValue :
- 33
- stepSize :
- 108
- numberOfNoiseLevelValues :
- 99
- xpath :
- /product/sourceAttributes/radarParameters/referenceNoiseLevel/noiseLevelValues
- noiseLevelValues_units :
- dB
- Description :
- Estimated noise level values as a function of georeferenced image pixel position in range. For SSG and SPG products, these apply to intermediate georeferenced image data prior to geocoding. (units = dB)
array([-26.5146694, -28.2042599, -28.9274807, -29.0195599, -28.6963501, -28.3008308, -28.0219402, -28.0109501, -28.10182 , -28.1521893, -28.0850506, -27.8957806, -27.6890507, -27.5236206, -27.4822502, -27.5732803, -27.6936607, -27.7577496, -27.7441597, -27.6663494, -27.5231991, -27.3503704, -27.2099991, -27.1921101, -27.2788696, -27.4200706, -27.6654301, -27.8861809, -28.0692596, -28.0071602, -27.6579609, -26.8420906, -28.4802094, -28.7677402, -28.5514908, -27.9778099, -27.3986301, -26.8556404, -26.5616398, -26.7301693, -27.26968 , -27.9239101, -28.4710999, -28.7484493, -28.7003899, -28.3253593, -27.7533302, -27.1461906, -26.7044792, -26.6575699, -27.0035591, -27.6537495, -28.37253 , -28.9162102, -29.1776695, -29.0733891, -28.55233 , -27.6127491, -26.3476009, -26.3748093, -26.4330502, -26.6009998, -26.86693 , -27.1536293, -27.3964806, -27.5256901, -27.5341702, -27.3796291, -27.1243801, -26.8217392, -26.5138092, -26.2992802, -26.2276192, -26.2963505, -26.4375801, -26.5832901, -26.6875706, -26.6256695, -26.3607407, -28.0145607, -28.3094196, -28.3012695, -28.1015892, -27.7658005, -27.38274 , -27.0402298, -26.8074608, -26.7485104, -26.8669605, -27.1168499, -27.4370499, -27.7481995, -27.9734993, -28.0289593, -27.8591995, -27.4492702, -26.8484993, -25.9767704, -24.8187904])
<xarray.DatasetView> Size: 3kB Dimensions: (beam: 4, pole: 2, pixel: 99) Coordinates: * beam (beam) <U3 48B 'W1' 'W2' 'W30' 'S7' * pole (pole) <U2 16B 'VH' 'VV' * pixel (pixel) int64 792B 0 1 2 3 4 5 6 ... 92 93 94 95 96 97 98 Data variables: beta0_noise_lut (pixel) float64 792B -22.05 -23.83 ... -26.65 -25.52 sigma0_noise_lut (pixel) float64 792B -26.78 -28.48 -29.21 ... -27.84 -26.7 gamma0_noise_lut (pixel) float64 792B -26.51 -28.2 -28.93 ... -25.98 -24.82
noise_lut- beam: 4
- pole: 2
- pixel: 99
- beam(beam)<U3'W1' 'W2' 'W30' 'S7'
array(['W1', 'W2', 'W30', 'S7'], dtype='<U3')
- pole(pole)<U2'VH' 'VV'
array(['VH', 'VV'], dtype='<U2')
- pixel(pixel)int640 1 2 3 4 5 6 ... 93 94 95 96 97 98
array([ 0, 1, 2, 3, 4, 5, 6, 7, 8, 9, 10, 11, 12, 13, 14, 15, 16, 17, 18, 19, 20, 21, 22, 23, 24, 25, 26, 27, 28, 29, 30, 31, 32, 33, 34, 35, 36, 37, 38, 39, 40, 41, 42, 43, 44, 45, 46, 47, 48, 49, 50, 51, 52, 53, 54, 55, 56, 57, 58, 59, 60, 61, 62, 63, 64, 65, 66, 67, 68, 69, 70, 71, 72, 73, 74, 75, 76, 77, 78, 79, 80, 81, 82, 83, 84, 85, 86, 87, 88, 89, 90, 91, 92, 93, 94, 95, 96, 97, 98])
- pulsesReceivedPerDwell(beam)int6458 58 58 58
- xpath :
- /product/sourceAttributes/radarParameters/pulsesReceivedPerDwell
- Description :
- ScanSAR burst parameter for ScanSAR only. Number of pulses received and recorded per dwell for each beam. Beam attribute values are same as those used in the beams list.
array([58, 58, 58, 58])
- numberOfPulseIntervalsPerDwell(beam)int6465 66 65 67
- xpath :
- /product/sourceAttributes/radarParameters/numberOfPulseIntervalsPerDwell
- Description :
- ScanSAR burst parameter for ScanSAR only. Number of pulse transmission intervals per dwell for each beam. Beam attribute values are same as those used in the beams list.
array([65, 66, 65, 67])
- rank(beam)int647 8 7 9
- xpath :
- /product/sourceAttributes/radarParameters/rank
- Description :
- Rank, one entry per beam. The number of transmitted pulse repetition intervals between transmission and reception for each beam. Samples per echo line. Used to determine sample window length. Beam attribute values are same as those used in the beams list.
array([7, 8, 7, 9])
- settableGain(beam, pole)float64-1.0 -1.0 -1.0 ... -1.0 -1.0 -1.0
- xpath :
- /product/sourceAttributes/radarParameters/settableGain
- wing :
- Combined
- units :
- dB
- Description :
- Gain values used on the instrument. Gain values will be specified either in terms of polarization or wing, not both. Beam attribute values are same as those used in the beams list. (units = dB). Wing can be either fore or aft.
array([[-1.00000002, -1.00000002], [-1.00000002, -1.00000002], [-1.00000002, -1.00000002], [-1.00000002, -1.00000002]])
- pulseRepetitionFrequency(beam)float641.272e+03 1.33e+03 ... 1.284e+03
- xpath :
- /product/sourceAttributes/radarParameters/pulseRepetitionFrequency
- units :
- Hz
- Description :
- Transmitted pulse repetition frequency for each beam. Beam attribute values are same as those used in the beams list (units = Hz). For products with two wing identifiers (Fore and Aft), the received PRF is effectively double the transmitted PRF.
array([1271.88439941, 1329.55175781, 1070.65319824, 1284.26281738])
- samplesPerEchoLine(beam)int646768 7920 7944 7320
- xpath :
- /product/sourceAttributes/radarParameters/samplesPerEchoLine
- Description :
- Number of samples per echo line for each beam. Used to determine sample window length. Beam attribute values are same as those used in the beams list.
array([6768, 7920, 7944, 7320])
- acquisitionType :
- ScanSAR Wide
- beams :
- ['W1', 'W2', 'W30', 'S7']
- polarizations :
- ['VV', 'VH']
- pulses :
- 11.58
- radarCenterFrequency_units :
- Hz
- radarCenterFrequency :
- 5404999242.769673
- pulseLength_pulse :
- 11.58
- pulseLength_units :
- s
- pulseLength :
- 4.152204895019531e-05
- pulseBandwidth_pulse :
- 11.58
- pulseBandwidth_units :
- Hz
- pulseBandwidth :
- 11597107.86
- antennaPointing :
- Right
- adcSamplingRate_pulse :
- 11.58
- adcSamplingRate_units :
- Hz
- adcSamplingRate :
- 12667968.75
- yawSteeringFlag :
- YawSteeringOn
- geodeticFlag :
- Off-Geocentric
- rawBitsPerSample :
- 4
- Description :
- Radar Parameters Data Store. Information describing the characteristics of the sensor used to acquire the data
- history :
- annotations
<xarray.DatasetView> Size: 1kB Dimensions: (beam: 4, pole: 2, pixel: 99) Coordinates: * beam (beam) <U3 48B 'W1' 'W2' 'W30' 'S7' * pole (pole) <U2 16B 'VH' 'VV' * pixel (pixel) int64 792B 0 1 2 3 4 ... 95 96 97 98 Data variables: pulsesReceivedPerDwell (beam) int64 32B 58 58 58 58 numberOfPulseIntervalsPerDwell (beam) int64 32B 65 66 65 67 rank (beam) int64 32B 7 8 7 9 settableGain (beam, pole) float64 64B -1.0 -1.0 ... -1.0 pulseRepetitionFrequency (beam) float64 32B 1.272e+03 ... 1.284e+03 samplesPerEchoLine (beam) int64 32B 6768 7920 7944 7320 Attributes: (12/21) acquisitionType: ScanSAR Wide beams: ['W1', 'W2', 'W30', 'S7'] polarizations: ['VV', 'VH'] pulses: 11.58 radarCenterFrequency_units: Hz radarCenterFrequency: 5404999242.769673 ... ... adcSamplingRate: 12667968.75 yawSteeringFlag: YawSteeringOn geodeticFlag: Off-Geocentric rawBitsPerSample: 4 Description: Radar Parameters Data Store. Information des... history: annotations
radarParameters- timeStamp: 11
- timeStamp(timeStamp)datetime64[ns]2022-04-07T18:21:27.401672 ... 2...
array(['2022-04-07T18:21:27.401672000', '2022-04-07T18:21:35.064601000', '2022-04-07T18:21:42.727530000', '2022-04-07T18:21:50.390460000', '2022-04-07T18:21:58.053389000', '2022-04-07T18:22:05.716318000', '2022-04-07T18:22:13.379247000', '2022-04-07T18:22:21.042176000', '2022-04-07T18:22:28.705106000', '2022-04-07T18:22:36.368035000', '2022-04-07T18:22:44.030964000'], dtype='datetime64[ns]')
- yaw(timeStamp)float643.678 3.667 3.659 ... 3.581 3.562
- units :
- deg
- xpath :
- /product/sourceAttributes/orbitAndAttitude/attitudeInformation/attitudeAngles/yaw
- Description :
- Spacecraft yaw angle (units = deg). Positive for nose right rotation about the z axis (i.e. clockwise when looking along the z axis). When Right-Looking, a small positive yaw generally means that the SAR antenna is pointing slightly backwards. When Left-Looking, a small positive yaw generally means it is pointing slightly forwards.
array([3.67765042, 3.66717625, 3.65942538, 3.64531175, 3.63299634, 3.62040748, 3.61178023, 3.60112808, 3.5906719 , 3.58090494, 3.56198898])
- roll(timeStamp)float64-29.8 -29.8 -29.8 ... -29.8 -29.8
- units :
- deg
- xpath :
- /product/sourceAttributes/orbitAndAttitude/attitudeInformation/attitudeAngles/roll
- Description :
- Spacecraft roll angle (units = deg). Positive for left side up rotation about the x axis (i.e. clockwise when looking along the x axis). Roll is negative for Right-Looking and positive for Left-Looking.
array([-29.8026275 , -29.8008087 , -29.80446062, -29.8055 , -29.8016028 , -29.79519935, -29.79506787, -29.7979482 , -29.80118764, -29.80025088, -29.79991269])
- pitch(timeStamp)float64-0.002003 -0.0003909 ... -0.001624
- units :
- deg
- xpath :
- /product/sourceAttributes/orbitAndAttitude/attitudeInformation/attitudeAngles/pitch
- Description :
- Spacecraft pitch angle (units = deg). Positive for nose up rotation about the y axis (i.e. clockwise when looking along the y axis).
array([-0.00200347, -0.00039085, -0.00467112, -0.0033236 , -0.00128973, 0.00014758, -0.00418872, -0.00425894, -0.00493375, -0.00499123, -0.00162387])
- xPosition(timeStamp)float64-6.653e+06 -6.632e+06 ... -6.42e+06
- units :
- m
- xpath :
- /product/imageAttributes/geographicInformation/geolocationGrid/imageTiePoint/stateVector/xPosition
- Description :
- (units = m)
array([-6653406.85785607, -6632011.72209174, -6610187.41538696, -6587935.6440859 , -6565258.00912605, -6542155.57507657, -6518630.37436722, -6494683.60325393, -6470317.51295421, -6445533.39145136, -6420333.0962503 ])
- yPosition(timeStamp)float641.034e+06 1.043e+06 ... 1.126e+06
- units :
- m
- xpath :
- /product/imageAttributes/geographicInformation/geolocationGrid/imageTiePoint/stateVector/yPosition
- Description :
- (units = m)
array([1033831.83554106, 1043489.20598069, 1053056.57779533, 1062532.90469045, 1071917.12401067, 1081208.16934229, 1090405.02030899, 1099506.52926137, 1108511.59959665, 1117419.48739412, 1126228.81609326])
- zPosition(timeStamp)float64-2.479e+06 -2.532e+06 ... -3e+06
- units :
- m
- xpath :
- /product/imageAttributes/geographicInformation/geolocationGrid/imageTiePoint/stateVector/zPosition
- Description :
- (units = m)
array([-2479415.41166767, -2532262.13585845, -2584948.0986012 , -2637469.66542779, -2689823.69039908, -2742006.69696019, -2794015.57314536, -2845846.87916092, -2897497.26875316, -2948963.70920172, -3000242.74537346])
- xVelocity(timeStamp)float642.764e+03 2.82e+03 ... 3.316e+03
- units :
- m/s
- xpath :
- /product/imageAttributes/geographicInformation/geolocationGrid/imageTiePoint/stateVector/xVelocity
- Description :
- (units = m/s)
array([2763.95730707, 2820.0484796 , 2875.94545209, 2931.64502427, 2987.14307326, 3042.4364157 , 3097.52119183, 3152.39354088, 3207.05004241, 3261.48723633, 3315.70149995])
- yVelocity(timeStamp)float641.266e+03 1.254e+03 ... 1.143e+03
- units :
- m/s
- xpath :
- /product/imageAttributes/geographicInformation/geolocationGrid/imageTiePoint/stateVector/yVelocity
- Description :
- (units = m/s)
array([1266.09239776, 1254.42208752, 1242.61059529, 1230.65867315, 1218.56751031, 1206.3380901 , 1193.97141416, 1181.46846028, 1168.83016818, 1156.05786562, 1143.15242659])
- zVelocity(timeStamp)float64-6.907e+03 ... -6.679e+03
- units :
- m/s
- xpath :
- /product/imageAttributes/geographicInformation/geolocationGrid/imageTiePoint/stateVector/zVelocity
- Description :
- (units = m/s)
array([-6906.77410476, -6885.99045794, -6864.77024034, -6843.1146525 , -6821.02546033, -6798.50404576, -6775.55188668, -6752.17053985, -6728.36180567, -6704.12638433, -6679.46673972])
- attitudeDataSource :
- Downlink
- attitudeOffsetsApplied :
- true
- Description :
- Attitude Information Data Store. Orbit Information Data Store. State Vector Data Store. Earth Centered Rotating (ECR) coordinates.
- history :
- annotations
<xarray.DatasetView> Size: 880B Dimensions: (timeStamp: 11) Coordinates: * timeStamp (timeStamp) datetime64[ns] 88B 2022-04-07T18:21:27.401672 ... ... Data variables: yaw (timeStamp) float64 88B 3.678 3.667 3.659 ... 3.591 3.581 3.562 roll (timeStamp) float64 88B -29.8 -29.8 -29.8 ... -29.8 -29.8 -29.8 pitch (timeStamp) float64 88B -0.002003 -0.0003909 ... -0.001624 xPosition (timeStamp) float64 88B -6.653e+06 -6.632e+06 ... -6.42e+06 yPosition (timeStamp) float64 88B 1.034e+06 1.043e+06 ... 1.126e+06 zPosition (timeStamp) float64 88B -2.479e+06 -2.532e+06 ... -3e+06 xVelocity (timeStamp) float64 88B 2.764e+03 2.82e+03 ... 3.316e+03 yVelocity (timeStamp) float64 88B 1.266e+03 1.254e+03 ... 1.143e+03 zVelocity (timeStamp) float64 88B -6.907e+03 -6.886e+03 ... -6.679e+03 Attributes: attitudeDataSource: Downlink attitudeOffsetsApplied: true Description: Attitude Information Data Store. Orbit Informati... history: annotations
orbitAndAttitude- timeOfDopplerCentroidEstimate: 6
- n-Coefficients: 5
- timeOfDopplerCentroidEstimate(timeOfDopplerCentroidEstimate)datetime64[ns]2022-04-07T18:21:27.475000 ... 2...
array(['2022-04-07T18:21:27.475000000', '2022-04-07T18:21:42.428868000', '2022-04-07T18:21:57.382736000', '2022-04-07T18:22:12.336603000', '2022-04-07T18:22:27.290472000', '2022-04-07T18:22:42.244341000'], dtype='datetime64[ns]')
- n-Coefficients(n-Coefficients)int640 1 2 3 4
array([0, 1, 2, 3, 4])
- dopplerAmbiguity(timeOfDopplerCentroidEstimate)int640 0 0 0 0 0
- xpath :
- /product/imageGenerationParameters/dopplerCentroid/dopplerAmbiguity
- Description :
- Doppler ambiguity number used during processing
array([0, 0, 0, 0, 0, 0])
- dopplerAmbiguityConfidence(timeOfDopplerCentroidEstimate)float640.9962 0.9962 ... 0.9962 0.9962
- xpath :
- /product/imageGenerationParameters/dopplerCentroid/dopplerAmbiguityConfidence
- Description :
- Doppler ambiguity confidence estimate.Range from 0.0 (no confidence) to 1.0 (highest confidence)
array([0.99622571, 0.99622571, 0.99622571, 0.99622571, 0.99622571, 0.99622571])
- dopplerCentroidReferenceTime(timeOfDopplerCentroidEstimate)float640.005613 0.005613 ... 0.005626
- units :
- s
- xpath :
- /product/imageGenerationParameters/dopplerCentroid/dopplerCentroidReferenceTime
- Description :
- 2-way slant range time used as reference in Doppler Centroid polynomial calculation (t0) (units = s)
array([0.00561258, 0.00561258, 0.00561258, 0.00561258, 0.00561258, 0.00562584])
- dopplerCentroidPolynomialPeriod(timeOfDopplerCentroidEstimate)float640.002049 0.002049 ... 0.002049
- units :
- s
- xpath :
- /product/imageGenerationParameters/dopplerCentroid/dopplerCentroidPolynomialPeriod
- Description :
- Approximate 2-way slant range time period in seconds corresponding to the slant range swath width. This is the period over which the Doppler centroid polynomial is valid, measured from Doppler centroid reference time (t0). For ScanSAR, this is the time corresponding to the full combined swath width.(units = s).
array([0.00204911, 0.00204911, 0.00204911, 0.00204911, 0.00204911, 0.00204911])
- dopplerCentroidCoefficients(timeOfDopplerCentroidEstimate, n-Coefficients)float64-196.1 7.52e+03 ... -2.112e+12
- xpath :
- /product/imageGenerationParameters/dopplerCentroid/dopplerCentroidCoefficients
- Description :
- List of up to 5 Doppler Centroid coefficients d0, d1, d2, d3, and d4 as a function of slant range timetSR where the Doppler Centroid frequency used in processing in Hz = d0 + d1(tSR - t0) + d2(tSR-t0)^2 + d3(tSR-t0)^3 + d4(tSR-t0)^4
array([[-1.96066406e+02, 7.52036621e+03, 7.81078700e+06, -5.08146022e+09, 1.86584098e+12], [-1.89800995e+02, 2.15785059e+04, -7.52412720e+07, 7.21335910e+10, -1.90014198e+13], [-2.14564896e+02, 1.66783188e+05, -1.88270304e+08, 3.63982111e+10, 1.26259298e+13], [-1.56520798e+02, -2.58028203e+05, 5.21606784e+08, -3.69894490e+11, 8.68939496e+13], [-1.99715393e+02, 1.50350266e+05, -1.87946704e+08, 8.88099717e+10, -1.39787302e+13], [-2.06129807e+02, 2.29637234e+05, -2.53270496e+08, 7.98278779e+10, -2.11173599e+12]])
- dopplerCentroidConfidence(timeOfDopplerCentroidEstimate)float640.9962 0.9977 ... 0.9948 0.9938
- xpath :
- /product/imageGenerationParameters/dopplerCentroid/dopplerCentroidConfidence
- Description :
- Doppler Centroid confidence estimate.Range from 0.0 (no confidence) to 1.0 (highest confidence)
array([0.99622571, 0.99767971, 0.99097651, 0.98627383, 0.99484169, 0.99383807])
- Description :
- Doppler Centroid Data Store
- history :
- annotations
<xarray.DatasetView> Size: 568B Dimensions: (timeOfDopplerCentroidEstimate: 6, n-Coefficients: 5) Coordinates: * timeOfDopplerCentroidEstimate (timeOfDopplerCentroidEstimate) datetime64[ns] 48B ... * n-Coefficients (n-Coefficients) int64 40B 0 1 2 3 4 Data variables: dopplerAmbiguity (timeOfDopplerCentroidEstimate) int64 48B ... dopplerAmbiguityConfidence (timeOfDopplerCentroidEstimate) float64 48B ... dopplerCentroidReferenceTime (timeOfDopplerCentroidEstimate) float64 48B ... dopplerCentroidPolynomialPeriod (timeOfDopplerCentroidEstimate) float64 48B ... dopplerCentroidCoefficients (timeOfDopplerCentroidEstimate, n-Coefficients) float64 240B ... dopplerCentroidConfidence (timeOfDopplerCentroidEstimate) float64 48B ... Attributes: Description: Doppler Centroid Data Store history: annotations
dopplerCentroid- dopplerRateReferenceTime: 1
- n-RateValuesCoefficients: 3
- dopplerRateReferenceTime(dopplerRateReferenceTime)float640.005613
array([0.005613])
- n-RateValuesCoefficients(n-RateValuesCoefficients)int640 1 2
array([0, 1, 2])
- dopplerRateValues(dopplerRateReferenceTime, n-RateValuesCoefficients)float64-2.162e+03 3.594e+05 -3.91e+07
- RateReferenceTime units :
- s
- dopplerRateReferenceTime_xpath :
- /product/imageGenerationParameters/dopplerRateValues/dopplerRateReferenceTime
- dopplerRateValuesCoefficients_xpath :
- /product/imageGenerationParameters/dopplerRateValues/dopplerRateValuesCoefficients
- Description :
- List of up to 5 Doppler rate values coefficients r0, r1, r2, r3, and r4 as a function of slant range time tSR where the Doppler frequency rate (in Hz per second of zero Doppler time) = r0 + r1(tSR - t0) + r2(tSR-t0)^2 + r3(tSR-t0)^3 + r4(tSR-t0)^4
array([[-2.16211890e+03, 3.59370219e+05, -3.90975000e+07]])
- Description :
- Doppler Rate Values Data Store.
- history :
- annotations
<xarray.DatasetView> Size: 56B Dimensions: (dopplerRateReferenceTime: 1, n-RateValuesCoefficients: 3) Coordinates: * dopplerRateReferenceTime (dopplerRateReferenceTime) float64 8B 0.005613 * n-RateValuesCoefficients (n-RateValuesCoefficients) int64 24B 0 1 2 Data variables: dopplerRateValues (dopplerRateReferenceTime, n-RateValuesCoefficients) float64 24B ... Attributes: Description: Doppler Rate Values Data Store. history: annotations
dopplerRateValues
<xarray.DatasetView> Size: 0B Dimensions: () Data variables: *empty*
doppler- pole: 2
- n-amplitudeCoefficients: 4
- n-phaseCoefficients: 4
- pole(pole)<U2'VV' 'VH'
- type :
- rs2prod:polarizationIdentifiers
array(['VV', 'VH'], dtype='<U2')
- n-amplitudeCoefficients(n-amplitudeCoefficients)int640 1 2 3
array([0, 1, 2, 3])
- n-phaseCoefficients(n-phaseCoefficients)int640 1 2 3
array([0, 1, 2, 3])
- replicaQualityValid(pole)<U4'true' 'true'
- xpath :
- /product/imageGenerationParameters/chirp/chirpQuality/replicaQualityValid
- Description :
- true = able to reconstruct all chirps or chirp reconstruction not requested (nominal chirp used) and all quality measures were acceptablefalse = unable to reconstruct chirp during processing and chirp reconstruction was requested or the quality is below acceptable levels.
array(['true', 'true'], dtype='<U4')
- crossCorrelationWidth(pole)float641.037 1.038
- xpath :
- /product/imageGenerationParameters/chirp/chirpQuality/crossCorrelationWidth
- Description :
- 3-dB pulse width of chirp replica cross-correlation function between reconstructed chirp and nominal chirp (units = samples)
array([1.03703594, 1.03756905])
- sideLobeLevel(pole)float64-13.33 -13.26
- xpath :
- /product/imageGenerationParameters/chirp/chirpQuality/sideLobeLevel
- units :
- dB
- Description :
- First side lobe level of chirp replica cross-correlation function between reconstructed chirp and nominal chirp (units = dB)
array([-13.32911968, -13.25835991])
- integratedSideLobeRatio(pole)float64-9.976 -10.16
- xpath :
- /product/imageGenerationParameters/chirp/chirpQuality/integratedSideLobeRatio
- units :
- dB
- Description :
- Integrated Side-Lobe Ratio of chirp replica cross-correlation function between reconstructed chirp and nominal chirp (units = dB)
array([ -9.97572708, -10.15859032])
- crossCorrelationPeakLoc(pole)float6438.61 38.21
- xpath :
- /product/imageGenerationParameters/chirp/chirpQuality/crossCorrelationPeakLoc
- Description :
- Cross correlation peak location. (units = samples)
array([38.60583496, 38.21051025])
- chirpPower(pole)float6464.28 64.17
- xpath :
- /product/imageGenerationParameters/chirp/chirpPower
- units :
- dB
- Description :
- (units = dB)
array([64.28054086, 64.16927446])
- amplitudeCoefficients(pole, n-amplitudeCoefficients)float641.0 -652.1 ... -2.642e+12
- xpath :
- /product/imageGenerationParameters/chirp/amplitudeCoefficients
- Description :
- List of range chirp amplitude coefficients (-, s^-1, s^-2, s^-3, ...)
array([[ 1.00000000e+00, -6.52071106e+02, 8.37702400e+06, -2.62762004e+12], [ 1.00000000e+00, -9.18793274e+02, -6.49115200e+07, -2.64203089e+12]])
- phaseCoefficients(pole, n-phaseCoefficients)float640.2689 1.349e+04 ... -1.012e+12
- xpath :
- /product/imageGenerationParameters/chirp/phaseCoefficients
- Description :
- List of range chirp phase coefficients (cycles, Hz, Hz/s, Hz/s^2, ...)
array([[ 2.68939286e-01, 1.34872969e+04, -1.39639505e+11, -5.13194787e+11], [ 8.32345009e-01, 4.97397266e+03, -1.39637899e+11, -1.01239901e+12]])
- VV_wing :
- Combined
- VV_pulse :
- 11.58
- VH_wing :
- Combined
- VH_pulse :
- 11.58
- Description :
- Chirp Data Store
- history :
- annotations
<xarray.DatasetView> Size: 320B Dimensions: (pole: 2, n-amplitudeCoefficients: 4, n-phaseCoefficients: 4) Coordinates: * pole (pole) <U2 16B 'VV' 'VH' * n-amplitudeCoefficients (n-amplitudeCoefficients) int64 32B 0 1 2 3 * n-phaseCoefficients (n-phaseCoefficients) int64 32B 0 1 2 3 Data variables: replicaQualityValid (pole) <U4 32B 'true' 'true' crossCorrelationWidth (pole) float64 16B 1.037 1.038 sideLobeLevel (pole) float64 16B -13.33 -13.26 integratedSideLobeRatio (pole) float64 16B -9.976 -10.16 crossCorrelationPeakLoc (pole) float64 16B 38.61 38.21 chirpPower (pole) float64 16B 64.28 64.17 amplitudeCoefficients (pole, n-amplitudeCoefficients) float64 64B 1.0 ... phaseCoefficients (pole, n-phaseCoefficients) float64 64B 0.2689 .... Attributes: VV_wing: Combined VV_pulse: 11.58 VH_wing: Combined VH_pulse: 11.58 Description: Chirp Data Store history: annotations
chirp
<xarray.DatasetView> Size: 0B Dimensions: () Data variables: *empty*
imageGenerationParameters- line: 513
- sample: 530
- pol: 2
- line(line)float649.5 29.5 ... 1.023e+04 1.025e+04
array([9.50000e+00, 2.95000e+01, 4.95000e+01, ..., 1.02095e+04, 1.02295e+04, 1.02495e+04])
- sample(sample)float649.5 29.5 ... 1.057e+04 1.059e+04
array([9.50000e+00, 2.95000e+01, 4.95000e+01, ..., 1.05495e+04, 1.05695e+04, 1.05895e+04])
- pol(pol)<U2'VV' 'VH'
array(['VV', 'VH'], dtype='<U2')
- digital_number(pol, line, sample)uint16dask.array<chunksize=(1, 513, 530), meta=np.ndarray>
- comment :
- not denoised digital number, resampled at "1000m" with rasterio.enums.Resampling.rms
- history :
- digital_number: imagery_V*.tif
Array Chunk Bytes 1.04 MiB 531.04 kiB Shape (2, 513, 530) (1, 513, 530) Dask graph 2 chunks in 3 graph layers Data type uint16 numpy.ndarray
<xarray.DatasetView> Size: 1MB Dimensions: (line: 513, sample: 530, pol: 2) Coordinates: * line (line) float64 4kB 9.5 29.5 49.5 ... 1.023e+04 1.025e+04 * sample (sample) float64 4kB 9.5 29.5 49.5 ... 1.057e+04 1.059e+04 * pol (pol) <U2 16B 'VV' 'VH' Data variables: digital_number (pol, line, sample) uint16 1MB dask.array<chunksize=(1, 513, 530), meta=np.ndarray>
digital_numbers- product_path :
- /home1/scratch/agrouaze/xsardatasync/xsardata/RS2_OK135107_PK1187782_DK1151894_SCWA_20220407_182127_VV_VH_SGF
- satellite :
- RADARSAT-2
- inputDatasetId :
- /Fred/RSAT-2/610044P
- rawDataStartTime :
- 2022-04-07T18:21:27.688416000
- satelliteHeight_units :
- m
- satelliteHeight :
- 800612.0083192665
- passDirection :
- Descending
- swath :
- SCWA
- product :
- SGF
- pols :
- VH VV
- name :
- RADARSAT2_DS:/home1/scratch/agrouaze/xsardatasync/xsardata/RS2_OK135107_PK1187782_DK1151894_SCWA_20220407_182127_VV_VH_SGF:
- start_date :
- 2022-04-07 18:21:27.401672
- stop_date :
- 2022-04-07 18:22:44.030964
- footprint :
- POLYGON ((168.8147284622835 -19.82390747944243, 163.9252572974075 -18.62823753782336, 162.6387987182235 -23.10230390032916, 167.6676039112585 -24.34397982283393, 168.8147284622835 -19.82390747944243))
- coverage :
- 515km * 530km (line * sample )
- pixel_line_m :
- 50.0
- pixel_sample_m :
- 50.0
- approx_transform :
- |-0.00,-0.00, 168.85| |-0.00, 0.00,-19.85| | 0.00, 0.00, 1.00|
How to apply calibration?
All the operation below are already performed by default for GRD products. So, what is following is a simple explanation about how is made calibration.
Load digital numbers
load_digital_number is a function that allows to load digital numbers from tiff files at chosen resolution and return it as a DataArray
. Resampling is made thanks to rasterio.open(tiff_file).read
. For dual pol products, there is a tiff file for each pol. So that digital numbers are computed for both pol. Posting of lines and samples is computed thanks to Affine.translation
and Affine.scale
.
[12]:
from xradarsat2 import load_digital_number
import rasterio
#Define resampling method (here it is the root mean square from rasterio)
resampling = rasterio.enums.Resampling.rms
#Define the chunks size for line and samples
chunks = {'line': 5000, 'sample': 5000}
[13]:
dn_low_res = load_digital_number(rs2ds.sar_meta.dt, resolution=resolution, resampling=resampling, chunks=chunks)['digital_numbers'].ds
dn_low_res
[13]:
<xarray.DatasetView> Size: 1MB Dimensions: (line: 513, sample: 530, pol: 2) Coordinates: * line (line) float64 4kB 9.5 29.5 49.5 ... 1.023e+04 1.025e+04 * sample (sample) float64 4kB 9.5 29.5 49.5 ... 1.057e+04 1.059e+04 * pol (pol) <U2 16B 'VV' 'VH' Data variables: digital_number (pol, line, sample) uint16 1MB dask.array<chunksize=(1, 513, 530), meta=np.ndarray>
- line: 513
- sample: 530
- pol: 2
- line(line)float649.5 29.5 ... 1.023e+04 1.025e+04
array([9.50000e+00, 2.95000e+01, 4.95000e+01, ..., 1.02095e+04, 1.02295e+04, 1.02495e+04])
- sample(sample)float649.5 29.5 ... 1.057e+04 1.059e+04
array([9.50000e+00, 2.95000e+01, 4.95000e+01, ..., 1.05495e+04, 1.05695e+04, 1.05895e+04])
- pol(pol)<U2'VV' 'VH'
array(['VV', 'VH'], dtype='<U2')
- digital_number(pol, line, sample)uint16dask.array<chunksize=(1, 513, 530), meta=np.ndarray>
- comment :
- not denoised digital number, resampled at "1000m" with rasterio.enums.Resampling.rms
- history :
- digital_number: imagery_V*.tif
Array Chunk Bytes 1.04 MiB 531.04 kiB Shape (2, 513, 530) (1, 513, 530) Dask graph 2 chunks in 3 graph layers Data type uint16 numpy.ndarray
- linePandasIndex
PandasIndex(Index([ 9.5, 29.5, 49.5, 69.5, 89.5, 109.5, 129.5, 149.5, 169.5, 189.5, ... 10069.5, 10089.5, 10109.5, 10129.5, 10149.5, 10169.5, 10189.5, 10209.5, 10229.5, 10249.5], dtype='float64', name='line', length=513))
- samplePandasIndex
PandasIndex(Index([ 9.5, 29.5, 49.5, 69.5, 89.5, 109.5, 129.5, 149.5, 169.5, 189.5, ... 10409.5, 10429.5, 10449.5, 10469.5, 10489.5, 10509.5, 10529.5, 10549.5, 10569.5, 10589.5], dtype='float64', name='sample', length=530))
- polPandasIndex
PandasIndex(Index(['VV', 'VH'], dtype='object', name='pol'))
Get the raw normalized radar cross section
_apply_calibration_lut
is a method that applies a calibration look up table to digital numbers to compute gamma0, beta0 and sigma0 (depending on the variable name passed in argument) and return the result as a DataArray
. It first get the high resolution calibration look up table. But it isn’t at the good resolution (already high resolution). So, this functions uses another one named _resample_lut_values
. Once the calibration look up table is at the good resolution, we can apply the
following formula :
Reference : Radarsat2 Product Format Definition
(7.2) : https://earth.esa.int/eogateway/documents/20142/0/Radarsat-2-Product-Format-Definition.pdf/1ca0cf1e-5a15-a29b-6187-9e5cb1650048#page=77
_resample_lut_values
uses RectBivariateSpline
for the interpolation, but it is necessary that data is expressed as a 2D vector. Here, calibration look up tables are expressed as 1D vector (range sample). Consequently, we need to convert these in 2D (adding an azimuth dimension dependency) before applying the interpolation. Conversion is made thanks to numpy.tile
, using the low resolution lines expressed in the geolocation grid part of the reader; reducing the calculation. A template
of a DataArray
that uses the posting of digital numbers (with applied resolution) is given on this interpolation function so the result is now at the right resolution.
Different resampling method were tried such as scipy.ndimage.gaussian_filter1d
that had the convenience to accept 1d vectors. Data was computed with this function and the chosen posting was this of digital numbers. But in order to be homogenous with other operations made in xsar
, we chose to keep the solution with RectBivariateSpline
.
[14]:
sigma0_raw = rs2ds._apply_calibration_lut('sigma0').sigma0_raw
sigma0_raw
[14]:
<xarray.DataArray 'sigma0_raw' (pol: 2, line: 513, sample: 530)> Size: 4MB dask.array<where, shape=(2, 513, 530), dtype=float64, chunksize=(1, 513, 530), chunktype=numpy.ndarray> Coordinates: * line (line) float64 4kB 9.5 29.5 49.5 ... 1.021e+04 1.023e+04 1.025e+04 * sample (sample) float64 4kB 9.5 29.5 49.5 ... 1.057e+04 1.059e+04 * pol (pol) <U2 16B 'VV' 'VH'
- pol: 2
- line: 513
- sample: 530
- dask.array<chunksize=(1, 513, 530), meta=np.ndarray>
Array Chunk Bytes 4.15 MiB 2.07 MiB Shape (2, 513, 530) (1, 513, 530) Dask graph 2 chunks in 20 graph layers Data type float64 numpy.ndarray - line(line)float649.5 29.5 ... 1.023e+04 1.025e+04
array([9.50000e+00, 2.95000e+01, 4.95000e+01, ..., 1.02095e+04, 1.02295e+04, 1.02495e+04])
- sample(sample)float649.5 29.5 ... 1.057e+04 1.059e+04
array([9.50000e+00, 2.95000e+01, 4.95000e+01, ..., 1.05495e+04, 1.05695e+04, 1.05895e+04])
- pol(pol)<U2'VV' 'VH'
array(['VV', 'VH'], dtype='<U2')
- linePandasIndex
PandasIndex(Index([ 9.5, 29.5, 49.5, 69.5, 89.5, 109.5, 129.5, 149.5, 169.5, 189.5, ... 10069.5, 10089.5, 10109.5, 10129.5, 10149.5, 10169.5, 10189.5, 10209.5, 10229.5, 10249.5], dtype='float64', name='line', length=513))
- samplePandasIndex
PandasIndex(Index([ 9.5, 29.5, 49.5, 69.5, 89.5, 109.5, 129.5, 149.5, 169.5, 189.5, ... 10409.5, 10429.5, 10449.5, 10469.5, 10489.5, 10509.5, 10529.5, 10549.5, 10569.5, 10589.5], dtype='float64', name='sample', length=530))
- polPandasIndex
PandasIndex(Index(['VV', 'VH'], dtype='object', name='pol'))
[15]:
import matplotlib.pyplot as plt
[16]:
plt.figure(figsize=(13, 6))
plt.subplot(1, 2, 1)
sigma0_raw_cross = sigma0_raw.sel(pol='VH')
plt.pcolor(sigma0_raw_cross, vmax=0.02, cmap='Greys_r')
plt.title('sigma0_raw VH')
plt.xlabel('samples')
plt.ylabel('lines')
plt.colorbar()
plt.subplot(1, 2, 2)
sigma0_raw_co = sigma0_raw.sel(pol='VV')
plt.pcolor(sigma0_raw_co, vmax=0.7, cmap='Greys_r')
plt.title('sigma0_raw VV')
plt.xlabel('samples')
plt.ylabel('lines')
plt.colorbar()
/tmp/ipykernel_1494600/739045657.py:9: MatplotlibDeprecationWarning: Getting the array from a PolyQuadMesh will return the full array in the future (uncompressed). To get this behavior now set the PolyQuadMesh with a 2D array .set_array(data2d).
plt.colorbar()
/tmp/ipykernel_1494600/739045657.py:17: MatplotlibDeprecationWarning: Getting the array from a PolyQuadMesh will return the full array in the future (uncompressed). To get this behavior now set the PolyQuadMesh with a 2D array .set_array(data2d).
plt.colorbar()
[16]:
<matplotlib.colorbar.Colorbar at 0x7c325c07c1d0>
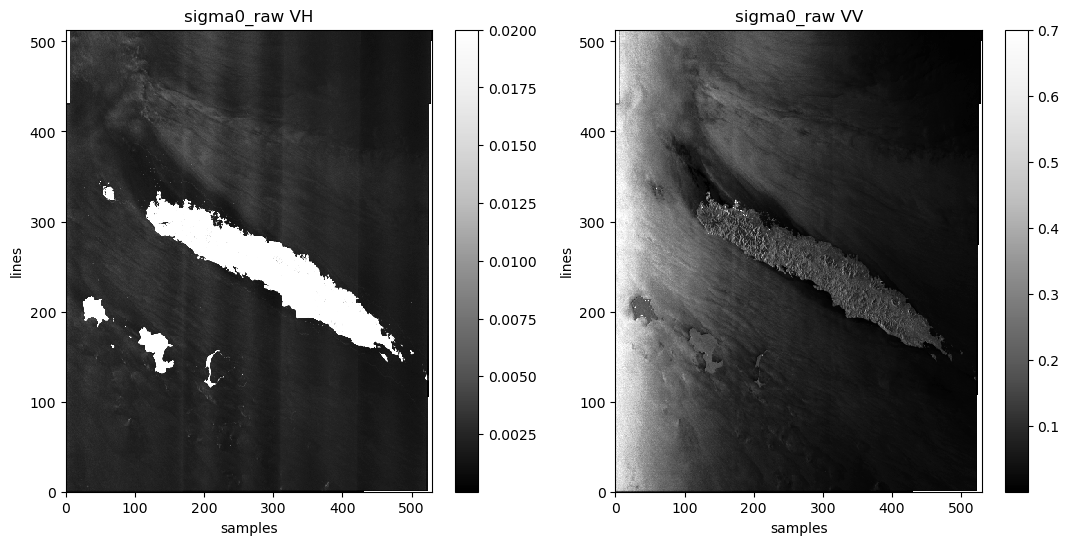
How to apply denoising ?
All the operation below are already performed by default for GRD products. So, what is following is a simple explanation about how is made denoising.
How to get the Noise Equivalent Sigma Zero ?
NoiseLevelValues
at low resolution are extracted from product.xml and then located in the datatree of the metadata (dt['radarParameters']
). They are already calibrated so we don’t have to apply a calibration on theseThey are expressed in dB
, and are given with a pixelFirstNoiseValue
and a stepSize
. With these information we have now to build the noise_lut
. The first thing to do is to convert the NoiseLevelValues in linear :
Right now we compute the nesz thanks to the following documentation : https://earth.esa.int/eogateway/documents/20142/0/Radarsat-2-Product-Format-Definition.pdf/1ca0cf1e-5a15-a29b-6187-9e5cb1650048#page=70
[17]:
nesz_low_res = rs2ds._interpolate_for_noise_lut('sigma0')
nesz_low_res
[17]:
<xarray.DataArray "Delayed('RectBivariateSpline-2bd80a40-1f1f-4d30-81-ba799a50b3ab57ddc3ccd8daa74d3243" ( line: 513, sample: 530)> Size: 1MB dask.array<Delayed('RectBivariateSpline-2bd80a40-1f1f-4d30-81, shape=(513, 530), dtype=float32, chunksize=(513, 530), chunktype=numpy.ndarray> Coordinates: * line (line) float64 4kB 9.5 29.5 49.5 ... 1.021e+04 1.023e+04 1.025e+04 * sample (sample) float64 4kB 9.5 29.5 49.5 ... 1.057e+04 1.059e+04
- line: 513
- sample: 530
- dask.array<chunksize=(513, 530), meta=np.ndarray>
Array Chunk Bytes 1.04 MiB 1.04 MiB Shape (513, 530) (513, 530) Dask graph 1 chunks in 5 graph layers Data type float32 numpy.ndarray - line(line)float649.5 29.5 ... 1.023e+04 1.025e+04
array([9.50000e+00, 2.95000e+01, 4.95000e+01, ..., 1.02095e+04, 1.02295e+04, 1.02495e+04])
- sample(sample)float649.5 29.5 ... 1.057e+04 1.059e+04
array([9.50000e+00, 2.95000e+01, 4.95000e+01, ..., 1.05495e+04, 1.05695e+04, 1.05895e+04])
- linePandasIndex
PandasIndex(Index([ 9.5, 29.5, 49.5, 69.5, 89.5, 109.5, 129.5, 149.5, 169.5, 189.5, ... 10069.5, 10089.5, 10109.5, 10129.5, 10149.5, 10169.5, 10189.5, 10209.5, 10229.5, 10249.5], dtype='float64', name='line', length=513))
- samplePandasIndex
PandasIndex(Index([ 9.5, 29.5, 49.5, 69.5, 89.5, 109.5, 129.5, 149.5, 169.5, 189.5, ... 10409.5, 10429.5, 10449.5, 10469.5, 10489.5, 10509.5, 10529.5, 10549.5, 10569.5, 10589.5], dtype='float64', name='sample', length=530))
[18]:
plt.pcolor(nesz_low_res, vmax=0.005, cmap='Greys_r')
plt.title('nesz')
plt.xlabel('samples')
plt.ylabel('lines')
plt.colorbar()
[18]:
<matplotlib.colorbar.Colorbar at 0x7c325c08a990>
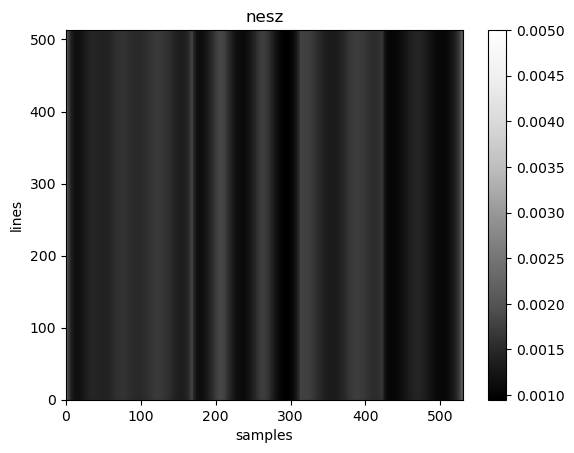
How to get the noise substracted Sigma0
Right now we only have to substract the noise_lut to the raw normalized radar cross section. It is made with the function _add_denoised
, that add the variables to the RadarSat2Dataset.dataset
[19]:
sigma0 = sigma0_raw - nesz_low_res
sigma0
[19]:
<xarray.DataArray (pol: 2, line: 513, sample: 530)> Size: 4MB dask.array<sub, shape=(2, 513, 530), dtype=float64, chunksize=(1, 513, 530), chunktype=numpy.ndarray> Coordinates: * line (line) float64 4kB 9.5 29.5 49.5 ... 1.021e+04 1.023e+04 1.025e+04 * sample (sample) float64 4kB 9.5 29.5 49.5 ... 1.057e+04 1.059e+04 * pol (pol) <U2 16B 'VV' 'VH'
- pol: 2
- line: 513
- sample: 530
- dask.array<chunksize=(1, 513, 530), meta=np.ndarray>
Array Chunk Bytes 4.15 MiB 2.07 MiB Shape (2, 513, 530) (1, 513, 530) Dask graph 2 chunks in 26 graph layers Data type float64 numpy.ndarray - line(line)float649.5 29.5 ... 1.023e+04 1.025e+04
array([9.50000e+00, 2.95000e+01, 4.95000e+01, ..., 1.02095e+04, 1.02295e+04, 1.02495e+04])
- sample(sample)float649.5 29.5 ... 1.057e+04 1.059e+04
array([9.50000e+00, 2.95000e+01, 4.95000e+01, ..., 1.05495e+04, 1.05695e+04, 1.05895e+04])
- pol(pol)<U2'VV' 'VH'
array(['VV', 'VH'], dtype='<U2')
- linePandasIndex
PandasIndex(Index([ 9.5, 29.5, 49.5, 69.5, 89.5, 109.5, 129.5, 149.5, 169.5, 189.5, ... 10069.5, 10089.5, 10109.5, 10129.5, 10149.5, 10169.5, 10189.5, 10209.5, 10229.5, 10249.5], dtype='float64', name='line', length=513))
- samplePandasIndex
PandasIndex(Index([ 9.5, 29.5, 49.5, 69.5, 89.5, 109.5, 129.5, 149.5, 169.5, 189.5, ... 10409.5, 10429.5, 10449.5, 10469.5, 10489.5, 10509.5, 10529.5, 10549.5, 10569.5, 10589.5], dtype='float64', name='sample', length=530))
- polPandasIndex
PandasIndex(Index(['VV', 'VH'], dtype='object', name='pol'))
Comparison between noised sigma0 and noised substracted sigma0
[20]:
plt.figure(figsize=(26, 12))
sigma0_cross = sigma0.sel(pol='VH')
sigma0_co = sigma0.sel(pol='VV')
plt.subplot(2,2,1)
plt.pcolor(sigma0_raw_cross, vmax=0.02, cmap='Greys_r')
plt.title('Sigma0 VH with noise')
plt.xlabel('samples')
plt.ylabel('lines')
plt.colorbar()
plt.subplot(2,2,3)
plt.pcolor(sigma0_cross, vmax=0.02, cmap='Greys_r')
plt.title('Sigma0 VH without noise')
plt.xlabel('samples')
plt.ylabel('lines')
plt.colorbar()
plt.subplot(2,2,2)
plt.pcolor(sigma0_raw_co, vmax=0.7, cmap='Greys_r')
plt.title('Sigma0 VV with noise')
plt.xlabel('samples')
plt.ylabel('lines')
plt.colorbar()
plt.subplot(2,2,4)
plt.pcolor(sigma0_co, vmax=0.7, cmap='Greys_r')
plt.title('Sigma0 VV without noise')
plt.xlabel('samples')
plt.ylabel('lines')
plt.colorbar()
/tmp/ipykernel_1494600/484210368.py:11: MatplotlibDeprecationWarning: Getting the array from a PolyQuadMesh will return the full array in the future (uncompressed). To get this behavior now set the PolyQuadMesh with a 2D array .set_array(data2d).
plt.colorbar()
/tmp/ipykernel_1494600/484210368.py:18: MatplotlibDeprecationWarning: Getting the array from a PolyQuadMesh will return the full array in the future (uncompressed). To get this behavior now set the PolyQuadMesh with a 2D array .set_array(data2d).
plt.colorbar()
/tmp/ipykernel_1494600/484210368.py:25: MatplotlibDeprecationWarning: Getting the array from a PolyQuadMesh will return the full array in the future (uncompressed). To get this behavior now set the PolyQuadMesh with a 2D array .set_array(data2d).
plt.colorbar()
/tmp/ipykernel_1494600/484210368.py:32: MatplotlibDeprecationWarning: Getting the array from a PolyQuadMesh will return the full array in the future (uncompressed). To get this behavior now set the PolyQuadMesh with a 2D array .set_array(data2d).
plt.colorbar()
[20]:
<matplotlib.colorbar.Colorbar at 0x7c32356a41d0>
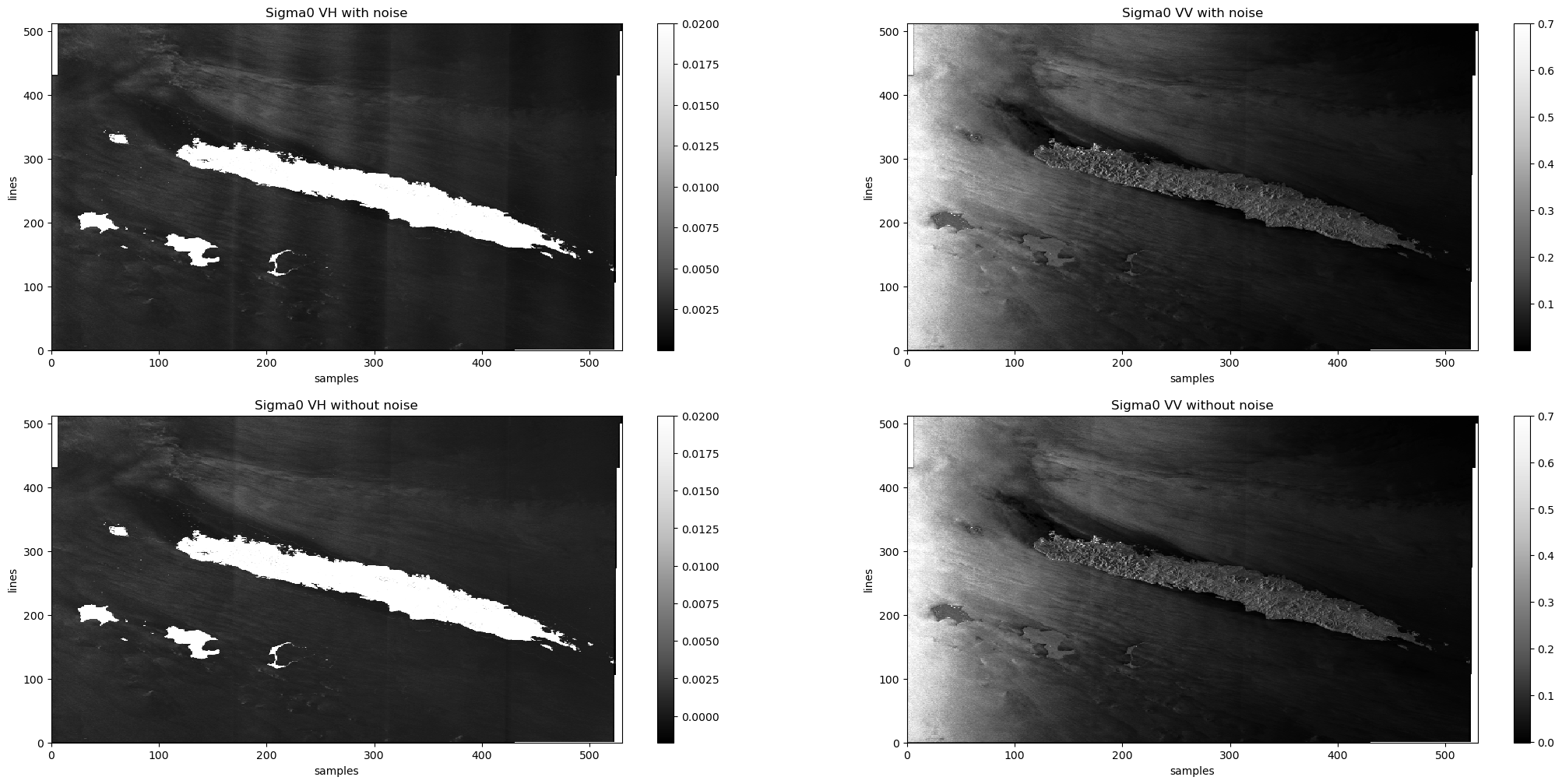
How to get the incidence ?
Radarsat2 product format definition (7.2) provides a formula of look up tables, depending on the incidence. We already have information about look up tables, so we determine incidence with these look up tables:
reference link : https://earth.esa.int/eogateway/documents/20142/0/Radarsat-2-Product-Format-Definition.pdf/1ca0cf1e-5a15-a29b-6187-9e5cb1650048#page=78
We have the choice between 2 types of look up tables: denoised and not denoised. We have chosen to determine incidence with not denoised look up tables. This computation is made by the function _load_incidence_from_lut
that returns a DatArray
[21]:
incidence = rs2ds._load_incidence_from_lut()
incidence
[21]:
<xarray.DataArray (line: 513, sample: 530)> Size: 2MB dask.array<degrees, shape=(513, 530), dtype=float64, chunksize=(513, 530), chunktype=numpy.ndarray> Coordinates: * line (line) float64 4kB 9.5 29.5 49.5 ... 1.021e+04 1.023e+04 1.025e+04 * sample (sample) float64 4kB 9.5 29.5 49.5 ... 1.057e+04 1.059e+04
- line: 513
- sample: 530
- dask.array<chunksize=(513, 530), meta=np.ndarray>
Array Chunk Bytes 2.07 MiB 2.07 MiB Shape (513, 530) (513, 530) Dask graph 1 chunks in 37 graph layers Data type float64 numpy.ndarray - line(line)float649.5 29.5 ... 1.023e+04 1.025e+04
array([9.50000e+00, 2.95000e+01, 4.95000e+01, ..., 1.02095e+04, 1.02295e+04, 1.02495e+04])
- sample(sample)float649.5 29.5 ... 1.057e+04 1.059e+04
array([9.50000e+00, 2.95000e+01, 4.95000e+01, ..., 1.05495e+04, 1.05695e+04, 1.05895e+04])
- linePandasIndex
PandasIndex(Index([ 9.5, 29.5, 49.5, 69.5, 89.5, 109.5, 129.5, 149.5, 169.5, 189.5, ... 10069.5, 10089.5, 10109.5, 10129.5, 10149.5, 10169.5, 10189.5, 10209.5, 10229.5, 10249.5], dtype='float64', name='line', length=513))
- samplePandasIndex
PandasIndex(Index([ 9.5, 29.5, 49.5, 69.5, 89.5, 109.5, 129.5, 149.5, 169.5, 189.5, ... 10409.5, 10429.5, 10449.5, 10469.5, 10489.5, 10509.5, 10529.5, 10549.5, 10569.5, 10589.5], dtype='float64', name='sample', length=530))
How to get the elevation ?
To get the incidence, we apply a formula :
Reference : Data Products Specifications (https://asf.alaska.edu/wp-content/uploads/2019/03/r1_prod_spec.pdf#page=47)
2 variables give orbit altitude so we considered theSatelliteHeight
(and not thealtitude
). RadarSat2Dataset._load_elevation_from_lut
permit to calculate the elevation (in degrees).
[22]:
elevation = rs2ds._load_elevation_from_lut()
elevation
[22]:
<xarray.DataArray (line: 513, sample: 530)> Size: 2MB dask.array<degrees, shape=(513, 530), dtype=float64, chunksize=(513, 530), chunktype=numpy.ndarray> Coordinates: * line (line) float64 4kB 9.5 29.5 49.5 ... 1.021e+04 1.023e+04 1.025e+04 * sample (sample) float64 4kB 9.5 29.5 49.5 ... 1.057e+04 1.059e+04
- line: 513
- sample: 530
- dask.array<chunksize=(513, 530), meta=np.ndarray>
Array Chunk Bytes 2.07 MiB 2.07 MiB Shape (513, 530) (513, 530) Dask graph 1 chunks in 43 graph layers Data type float64 numpy.ndarray - line(line)float649.5 29.5 ... 1.023e+04 1.025e+04
array([9.50000e+00, 2.95000e+01, 4.95000e+01, ..., 1.02095e+04, 1.02295e+04, 1.02495e+04])
- sample(sample)float649.5 29.5 ... 1.057e+04 1.059e+04
array([9.50000e+00, 2.95000e+01, 4.95000e+01, ..., 1.05495e+04, 1.05695e+04, 1.05895e+04])
- linePandasIndex
PandasIndex(Index([ 9.5, 29.5, 49.5, 69.5, 89.5, 109.5, 129.5, 149.5, 169.5, 189.5, ... 10069.5, 10089.5, 10109.5, 10129.5, 10149.5, 10169.5, 10189.5, 10209.5, 10229.5, 10249.5], dtype='float64', name='line', length=513))
- samplePandasIndex
PandasIndex(Index([ 9.5, 29.5, 49.5, 69.5, 89.5, 109.5, 129.5, 149.5, 169.5, 189.5, ... 10409.5, 10429.5, 10449.5, 10469.5, 10489.5, 10509.5, 10529.5, 10549.5, 10569.5, 10589.5], dtype='float64', name='sample', length=530))